Imagine you’re an analyst in 1895, leafing through a dusty ledger to decide whether to extend credit to a growing manufacturer—your next call could make or break the bank’s bottom line. Fast forward to today: you’re using AI-powered tools that process thousands of data points in seconds, highlighting trends and insights that once took hours to uncover manually. Yet the core question remains the same: How healthy is this business?
Key Takeaways
- The origin of financial statement analysis started as a way for banks to assess the creditworthiness of borrowers.
- Uniform accounting standards laid the groundwork for credible, repeatable financial evaluation.
- Modern tools combine time-tested approaches with financial data analysis techniques for better decisions.
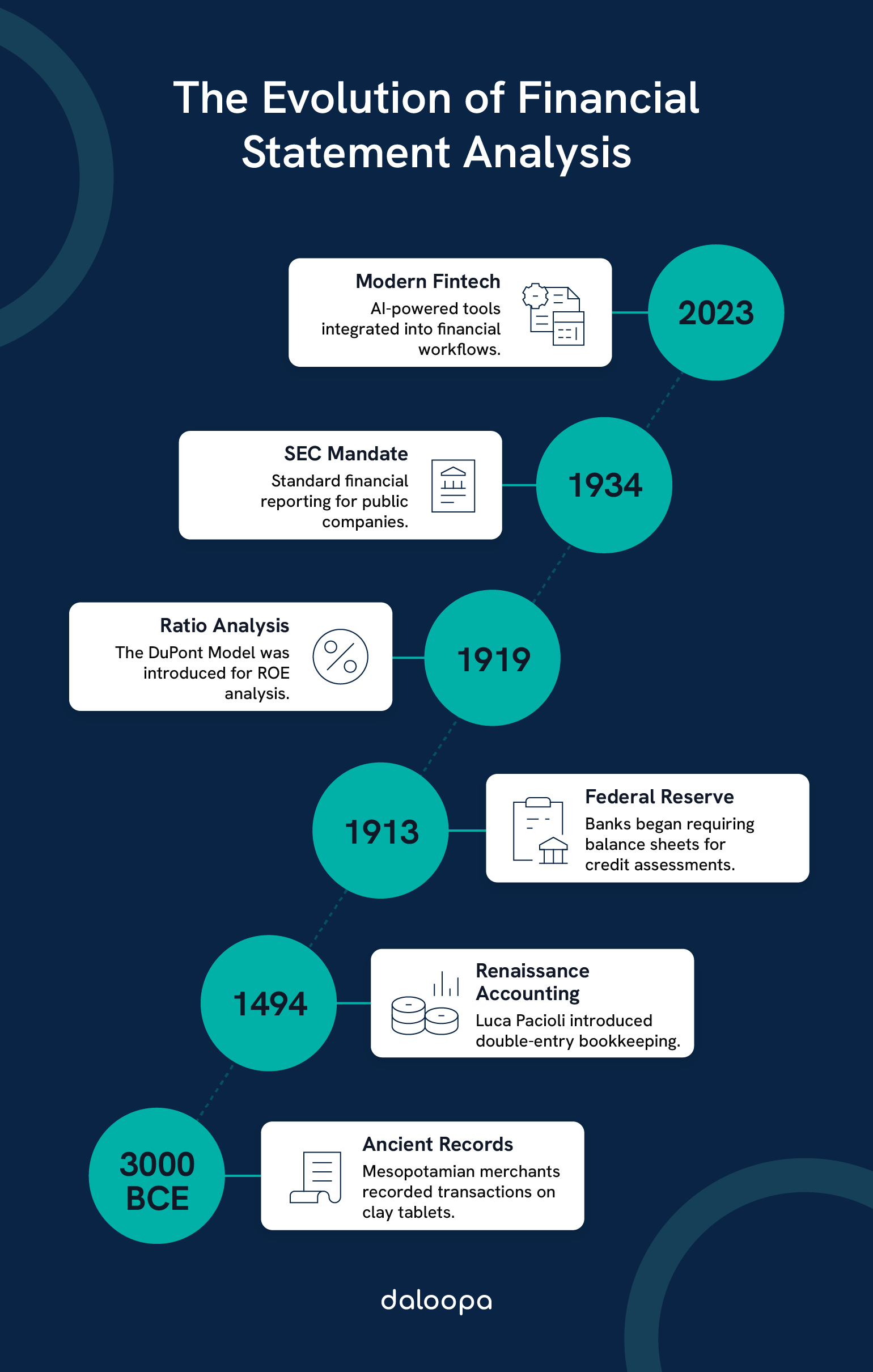
Ancient Foundations of Financial Statement Analysis
Long before banks in the 19th century formalized credit assessments, civilizations documented economic activity to manage resources and trade. These early practices laid the groundwork for the origin of financial statement analysis and today’s sophisticated analytical frameworks.
Record‑Keeping in Early Civilizations
Mesopotamian merchants etched transactions onto clay tablets around 3000 BCE, tracking livestock, grain, and labor. They used primitive “debit” and “credit” entries to ensure records were balanced—an early nod to the double‑entry logic analysts still rely on. Ancient Egyptians and Babylonians followed suit, maintaining temple and state ledgers that detailed assets, obligations, and tax collections. These documents formed the earliest financial data analysis techniques, allowing leaders to compare performance over time. Standardizing these entries enabled rulers and traders to compare harvest yields, loan obligations, and tax revenues over time—effectively performing horizontal and vertical analyses on their own terms.
Renaissance Revolution: Pacioli and the Birth of Modern Accounting
In 1494, Luca Pacioli published Summa de Arithmetica, introducing double‑entry bookkeeping to the merchant class in Venice. He defined “debito” (what one owes) and “credito” (what one entrusts), and outlined three core books—memorandum, journal, and ledger—that ensured every transaction left a balanced trail. This became a cornerstone in the origin of financial statement analysis.
Pacioli’s treatise spread rapidly across Europe, transforming bookkeeping from ad‑hoc record‑keeping into a structured discipline. Merchants and bankers could now perform ratio‑style comparisons and track profitability with unprecedented precision. This Renaissance leap echoes in today’s financial models, where every line item traces back to Pacioli’s insistence on balance and transparency.
The Origin Of Financial Statement Analysis
The origin of financial statement analysis evolved to meet the real‑world needs of investors, lenders, and regulators. Tracing its roots shows why modern frameworks still rest on century‑old pillars.
Early Beginnings In Financial Reporting
In the late 1800s, banks began requiring prospective borrowers to submit balance sheets detailing assets and liabilities. This shift moved lending decisions beyond intuition to evidence‑based evaluation. When Congress created the Federal Reserve in 1913, standardized banking practices pushed institutions to adopt more formal financial data analysis techniques, enhancing accuracy and comparability.
Modern credit committees still rely on historical financials to gauge a borrower’s resilience and runway—echoing those first balance sheet reviews.
The Evolution Of Financial Concepts
The 1920s ushered in ratio analysis, empowering analysts to compare companies of different sizes. The DuPont Model, devised in 1919 by Donaldson Brown at DuPont, broke return on equity (ROE) into profit margin, asset turnover, and financial leverage, offering a cause‑and‑effect view of performance.
The 1920s brought ratio analysis to life, helping analysts understand performance regardless of company size. As part of the origin of financial statement analysis, these tools helped shift focus from simple bookkeeping to strategic insights.
In 1934, the SEC mandated periodic reporting for public companies, compelling uniform disclosure of income statements and cash flow statements. These additions enabled deeper dives into operational performance and liquidity health.
Development Of Analytical Techniques
As businesses grew complex, analysts refined their tools. What began as simple comparisons transformed into structured methodologies that illuminate a company’s financial condition.
Introduction Of Ratio Analysis
Analysts first used liquidity ratios like the current ratio and working capital to assess short‑term stability. By the 1920s, profitability ratios (e.g., return on assets) joined the toolkit, enabling holistic performance views. Over decades, the list expanded to include leverage and efficiency metrics, turning ratios into diagnostic benchmarks.
Metrics like return on assets and current ratio turned numbers into signals. These ratios, among the earliest financial data analysis techniques, remain diagnostic tools used in tandem with modern financial report analysis software.
Horizontal And Vertical Analysis
Horizontal analysis compares line items over time, highlighting trends and anomalies, while vertical analysis expresses each item as a percentage of a base figure (e.g., revenue), normalizing size differences.
Horizontal trends reveal growth patterns or warning flags; vertical metrics enable cross‑company comparisons regardless of scale.
These techniques, dating back to early financial statement reviews, allowed for comparisons across time and between firms—core to the origin of financial statement analysis.
The DuPont Analysis
Introduced in 1919, the DuPont model broke down ROE and revealed performance drivers. It’s a legacy tool still embedded in today’s financial report analysis software.
The DuPont framework dissects ROE into three levers: profit margin, asset turnover, and financial leverage. By examining each, analysts can pinpoint drivers of return and areas for improvement. Although newer metrics (e.g., ROIC) have emerged, DuPont remains a foundational tool in business schools and boardrooms alike.
Influence Of Historical Foundations On Modern Practices
The frameworks and habits established over the last century still influence how financial statements are read and used. Analysts today build on these foundations with updated tools, but the core principles endure.
Informing Contemporary Financial Analysis
The roots of today’s analysis stretch back centuries. The double-entry bookkeeping method, introduced in the 1400s, is still the spine of modern accounting. Today, it also supports cloud-based financial report analysis software that facilitates real-time performance tracking. It ensures balance and structure, making financial data analyzable.
Ratios evolved from basic credit checks into comprehensive diagnostic tools. Though more refined, their purpose remains the same: measure how well a business operates and where its risks lie.
The push for standardized financial reporting in the late 1800s brought clarity to the field. That clarity allows for effective trend analysis—essential for comparing performance across time and sectors.
Lessons From The Past For Today’s Analysts
Early credit assessments prioritized thorough documentation and conservative assumptions—practices that risk managers still apply. Past crises, from the Panic of 1907 to the 2008 financial meltdown, taught analysts to blend quantitative metrics with qualitative context.
Watch For:
- Shrinking cash flows without clear drivers
- Margin compression unbacked by strategic shifts
- Debt spikes lacking revenue growth support
These red flags, first spotted in ledgers, remain vital signals in automated dashboards.
Reflecting On The Progress Of Financial Statement Analysis
Financial statement analysis mirrors the evolution of business and technology. What began as a ledger-based necessity has matured into a discipline powered by statistical modeling, real-time data, and AI-enhanced automation.
From Rule-Based Systems to AI Agents
The use of AI in financial analysis dates back to the 1980s and 1990s, when early rule-based systems and simple algorithms were introduced for trading strategies and basic risk management. These early tools used fixed logic and statistical methods to automate narrow tasks, laying the groundwork for more adaptive systems.
New Milestones in the 2010s and Beyond
In the 2010s, financial institutions and hedge funds began adopting emerging fintech tools powered by machine learning to improve forecasting, credit modeling, document classification, and risk assessment. These systems could process large volumes of financial documents and extract structured data, but often required significant manual setup, training, and oversight.
The early 2020s marked a turning point. The arrival of generative AI and large language models (LLMs) brought context-aware analysis, document summarization, and natural language interaction to the forefront. These tools made AI more accessible to analysts and transformed how unstructured financial data—like earnings calls, 10-Ks, and investor presentations—is understood and used.
By 2023, AI-powered fintech had become deeply integrated into financial workflows. LLMs and AI agents were widely used to automate research, detect anomalies, generate summaries, and support faster, real-time decision-making across the industry.
Daloopa and the Automation of Analyst Workflows
A standout in this new wave is Daloopa, a platform that uses AI to automatically extract financial data from SEC filings, earnings transcripts, and investor presentations. Every data point is mapped to its source, allowing analysts to audit and update models quickly while spending more time on interpretation and less on manual data entry. It represents a practical leap in efficiency, especially for investment analysts, equity researchers, and asset managers.
Other Modern Developments
- Unified reporting templates: Cloud-based models now standardize financial formats across teams and organizations, ensuring consistency and auditability.
- Decision‑support systems: Interactive tools powered by analytics and visualization help analysts perform scenario planning, stress testing, and forecasting in real time.
- Continuous learning: Online platforms, CFA programs, and professional certifications now incorporate analytics, modeling, and AI literacy into core finance education.
Today, financial analysts rely on AI and machine learning to uncover patterns, predict future performance, and reduce manual friction—advancements that were unimaginable just a decade ago. The field continues to evolve with technology, changing not just how financial data is reviewed, but how it’s interpreted and used to drive decisions.
Take Your Financial Statement Analysis to the Next Level
Whether you’re building models or analyzing performance, understanding the origin of financial statement analysis provides essential context for making sharper decisions. With Daloopa’s AI-powered platform, you can shift from time-consuming data gathering to focused, high-impact analysis. Automate the extraction of financial data, trace every figure back to its original source, and keep your models current with real-time updates. See how Daloopa can streamline your workflow and fuel your next big move.