Artificial intelligence significantly enhances efficiency and accuracy in financial institutions by reducing reliance on manual processes, enabling faster and more informed decision-making. As firms increasingly adopt AI solutions to leverage big data and optimize operations, they gain a competitive edge. AI-driven technologies further promote innovation by facilitating precise and rapid data analysis, driving operational improvements across the industry.
Key Takeaways
- AI improves efficiency and accuracy in financial data extraction.
- Financial services benefit from AI’s capacity for handling vast data.
- AI advancements promise further innovation in finance.
AI Technology in Financial Data Extraction
Advances in artificial intelligence (AI) are transforming data extraction within financial services, offering greater efficiency and precision in handling vast datasets. Machine learning (ML) automates data analysis and provides insights critical for investment decisions.
Foundations of AI and Machine Learning
AI and machine learning form the backbone of modern data extraction. AI technologies are designed to replicate human cognitive functions, enabling systems to autonomously perform tasks such as identifying patterns, categorizing data, and improving accuracy over time. Machine learning, a key component of AI, processes large volumes of structured and unstructured financial data, allowing models to continuously enhance performance.
For financial analysts, natural language processing (NLP) is particularly relevant, enabling efficient extraction and categorization of text-based data from documents such as earnings reports and contracts, accelerating analysis, and improving the accuracy of extracting key financial information.
Applications of AI in Financial Data Extraction
AI’s applications in financial data extraction span a wide range of functions. In areas such as risk assessment, AI-driven models analyze transaction patterns to detect anomalies, aiding in fraud detection and regulatory compliance. For example, AI can streamline the Know Your Customer (KYC) process by automating document verification, reducing manual errors, and speeding up onboarding.
Additionally, AI supports portfolio management by analyzing vast amounts of market data and extracting insights that inform investment strategies. In tools like Daloopa for instance, AI automates data extraction and provides accurate, structured data that helps analysts assess portfolio risks and optimize asset allocation based on market forecasts. These capabilities enable financial institutions to make more informed decisions and respond quickly to market changes, enhancing both the speed and accuracy of investment analyses.
Integrating AI into data extraction processes reduces operational costs through automation and enhances scalability, ensuring firms can handle increasing data volumes while maintaining accuracy and compliance.
The Strategic Role of Data in Finance
In the financial sector, data serves as a cornerstone for driving informed investment decisions, shaping strategies, and forecasting market trends. Analysts can build accurate models, mitigate risk, and optimize returns by harnessing the right financial data.
Financial Documents and Key Data Sources
Investment decisions begin with an in-depth analysis of core financial documents like balance sheets, income statements, and cash flow statements, which provide critical insights into a company’s financial health. Beyond these traditional documents, sophisticated analysts utilize market reports, transaction histories, and customer data to enrich their understanding of market dynamics and corporate performance.
These diverse data sources create a broader view of financial trends and company behavior, helping analysts assess profitability, manage risk, and predict market movements more precisely. For instance, tools like regression analysis enable deeper insights into historical performance, while Monte Carlo simulations offer probabilistic scenarios for future projections.
Leveraging Advanced Analytics for Financial Decisions
Integrating advanced analytics, such as machine learning algorithms in AI-driven tools like Daloopa, has redefined financial decision-making. These technologies allow analysts to turn vast datasets into actionable insights, driving smarter portfolio management and efficient risk assessment. AI can analyze data in real-time, adjusting portfolios dynamically based on changing market conditions.
By using these advanced techniques, financial institutions reduce uncertainty and improve strategic planning, enabling a data-driven approach that enhances the precision and robustness of investment decisions. Whether forecasting market trends or conducting variance analysis, data remains at the heart of every decision, ensuring that investments are backed by thorough, objective analysis.
Challenges of Manual Data Extraction in Financial Services
Manual data extraction in financial services presents several challenges, primarily affecting efficiency, accuracy, and scalability. These challenges are especially pronounced when processing large volumes of unstructured data, which increasingly characterize modern financial environments.
Data Entry Errors and Their Impact
Manual data entry is inherently prone to errors, such as typographical mistakes, misinterpretation of data, or simple oversight. In financial services, even minor errors can lead to significant miscalculations that affect portfolio valuations, risk assessments, and regulatory filings, increasing the risk of non-compliance with regulatory requirements leading to financial penalties or reputational damage.
For investment banking analysts, the need for precise and reliable data is paramount, especially when drawing insights from complex documents like financial statements or market reports. Manual methods often lack the necessary precision, resulting in increased operational costs and potential delays in decision-making. Automation, by contrast, can validate and process data in real time, reducing the likelihood of errors.
Limitations of Manual Processing
The limitations of manual data extraction become most apparent when handling large datasets or unstructured documents. Manual methods are time-consuming, labor-intensive, and difficult to scale, slowing down critical financial workflows, such as compiling reports from multiple data sources or conducting comprehensive risk assessments.
For instance, compiling earnings reports or manually analyzing large transaction histories can take days, while automated systems can process the same data in minutes, hindering the ability to react to market changes swiftly and reducing operational efficiency. AI-driven solutions provide a scalable and agile alternative, enabling faster, more accurate data extraction that keeps pace with the demands of modern financial operations.
Technological Innovations in Data Extraction
Innovations in data extraction technology have dramatically enhanced the efficiency of processing financial documents. By leveraging AI, these technologies significantly reduce manual workload and improve accuracy.
Optical Character Recognition (OCR) Advances
Optical Character Recognition (OCR) technology has made significant strides in enhancing data extraction and automation across the financial industry. Modern OCR systems, powered by machine learning techniques, can accurately process a wide range of document formats, including scanned images, PDFs, and handwritten text.
In financial services, OCR has become indispensable for tasks like Know Your Customer (KYC) compliance and fraud detection. For example, investment banks and fintech firms leverage AI-enhanced OCR to streamline the onboarding process by verifying identification documents quickly and accurately. Additionally, OCR’s integration with broader financial systems allows firms to automate compliance checks, ensuring that critical data from regulatory filings is processed accurately and efficiently.
Natural Language Processing (NLP) in Document Processing
Natural Language Processing (NLP) is transforming the way financial institutions process and analyze vast amounts of unstructured data. In the investment banking and hedge fund sectors, NLP is increasingly used to derive actionable insights, helping firms gain a competitive edge by extracting valuable information from sources like earnings calls, market news, and financial reports.
Daloopa, as an example, uses AI-driven natural language processing and machine learning to provide more accurate and comprehensive fundamental datasets, surpassing traditional vendors like FactSet and S&P CapIQ. The platform, which captures extensive non-GAAP data, automates data collection processes modeled on buy-side analyst workflows and offers access via Excel plug-ins and APIs.
- Contract Analysis: NLP algorithms streamline the analysis of complex legal documents by identifying key terms, clauses, and obligations, reducing the time spent manually reviewing contracts and ensuring compliance with regulatory requirements. For example, large investment banks use NLP to analyze loan agreements and derivative contracts, automatically flagging potential risks or violations of terms.
- Sentiment Analysis of Market News: Hedge funds and asset managers employ NLP to analyze market news, press releases, and social media sentiment. By gauging market sentiment, these firms can predict stock price movements or assess the impact of geopolitical events on their portfolios.
Earnings Calls and Reports: NLP is also used to extract key data from earnings calls and quarterly reports, enabling real-time analysis of a company’s performance and future outlook. NLP systems can detect nuanced language changes in CEOs’ or CFOs’ speeches, providing insights into market confidence or potential risks. Investment firms leverage this capability to refine their forecasting models and inform buy/sell decisions. By integrating NLP with machine learning algorithms, financial institutions can process and analyze large datasets with speed and accuracy. AI-based document processing has significantly improved financial services by improving accuracy, efficiency, and scalability. Machine learning models allow these systems to adapt and refine their capabilities continuously, leading to more precise data extraction and validation over time.
The scalability of AI-based systems also allows firms to manage increasing volumes of data without sacrificing speed or accuracy. These innovations enable more efficient, responsive, and adaptable financial operations, particularly in high-stakes environments where timely insights are critical.
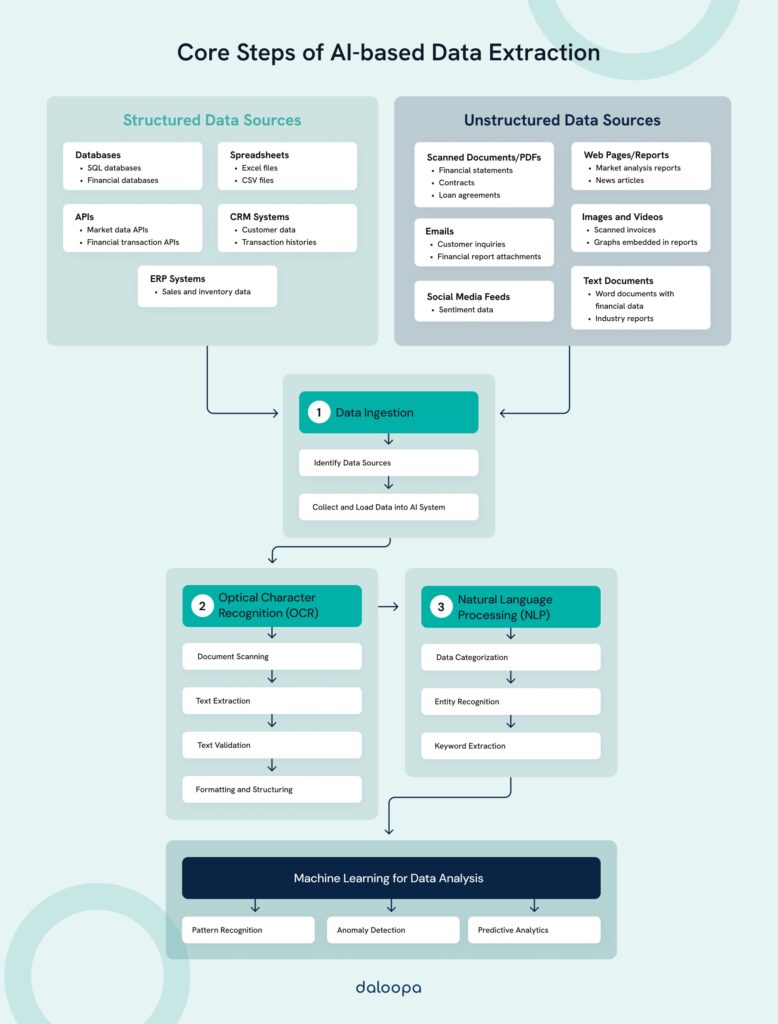
Quantifiable Advantages of AI-Based Data Extraction in Financial Services
AI-driven data extraction delivers measurable benefits across efficiency, accuracy, and regulatory compliance, directly impacting both operational costs and strategic decision-making in the financial sector.
Enhancing Efficiency and Scalability
AI systems significantly reduce the time and resources required for data extraction by automating manual processes. For example, tasks that typically take analysts hours—such as extracting financial data from SEC filings or processing large datasets—can now be completed in minutes. On average, firms report up to an 80% reduction in processing time, which translates to substantial cost savings in labor and operational expenses.
Furthermore, AI’s ability to scale is invaluable in high-volume environments, such as investment banking or asset management. As datasets grow, AI systems maintain performance without compromising speed or accuracy, supporting scalable operations without increasing staff costs.
Achieving High Accuracy and Regulatory Compliance
AI enhances the precision of financial data extraction, reducing errors commonly associated with manual data entry or traditional methods. For example, Research indicates that the use of AI in financial operations leads to a reduction in reporting errors by up to 37%. (Sarker, 2022)
In terms of regulatory compliance, AI tools are built to automatically update with new laws and regulations, ensuring that data handling aligns with evolving legal standards, reducing the need for frequent manual adjustments, and lowering the risk of non-compliance.
Implementation in Key Financial Sectors
AI automates processes and enhances data extraction, leading to improved operational efficiency and more robust data-driven insights.
Banking Sector Transformation
In the banking industry, AI systems automate transaction processing, anomaly detection, and fraud prevention, allowing institutions to handle vast data volumes efficiently. Machine learning models help predict customer behavior, enabling more personalized services and optimized marketing strategies. AI systems also streamline loan assessments by quickly analyzing customer profiles and credit scores, reducing decision times. These applications lead to enhanced operational efficiency, lower costs, and improved customer satisfaction.
AI in Insurance Data Management
AI-based systems significantly improve data management in the insurance sector by automating tasks such as document processing and claims handling, reducing manual errors, and speeding up workflows. Natural language processing (NLP) aids in managing customer inquiries and claims documentation more efficiently. In addition, AI-powered predictive analytics enable more accurate risk assessments and competitive pricing. AI systems also support underwriting by quickly evaluating client data and potential risks, allowing insurers to offer more tailored products.
Enhancements in Accounting and Auditing
AI improves accuracy and efficiency in accounting and auditing by automating routine tasks such as transaction categorization and data entry, allowing professionals to focus on higher-value activities. AI-driven tools analyze financial data for trends and anomalies, facilitating real-time financial reporting. AI systems also help firms stay up-to-date with regulatory changes, ensuring compliance with evolving standards.
Custom Solutions for Financial Data Extraction
When developing financial data extraction solutions, the focus is on creating adaptive, AI-powered software tailored to the specific needs of financial institutions. These solutions enhance the efficiency and accuracy of data processing within existing financial systems.
Building Customizable Data Extraction Software
The development of customizable financial data extraction tools centers on adaptability. These solutions are designed to integrate seamlessly with existing workflows, handling diverse data formats and sources with minimal manual intervention. Automation and intelligent document processing reduce errors while improving efficiency.
User-friendly software allows financial institutions to modify settings as their data needs evolve, ensuring that the software remains relevant and scalable and providing the ability to automate data extraction processes without extensive technical expertise.
Integrating AI with Existing Financial Systems
AI-based system integration significantly enhances the processing capabilities of existing financial systems by automating the extraction of large datasets and identifying critical patterns. These AI-driven algorithms enable real-time data analysis, supporting quicker and more informed decision-making.
Additionally, AI improves data security by streamlining extraction processes and safeguarding sensitive information. The integration is designed to maintain operational continuity while boosting system efficiency, ensuring compatibility with diverse financial platforms, and facilitating smooth transitions.
Future Trends in AI Data Extraction
The evolution of AI-based data extraction is set to significantly impact the financial industry, transforming data processing methodologies and enhancing efficiency and accuracy, enabling financial institutions to manage large volumes of data seamlessly, facilitating insightful analysis and informed strategic decision-making.
Market Growth
The data extraction market is experiencing substantial growth, driven by AI advancements that enhance accessibility and functionality. Financial institutions increasingly adopt these technologies to extract relevant information quickly, responding to the rising demand for real-time data insights.
Key Trends
- Increased Automation: The automation of data extraction processes reduces human error and accelerates the speed of data processing, enabling financial firms to operate more efficiently.
- Scalable Solutions: As data volumes expand, scalable AI-based solutions allow financial companies to manage vast datasets without compromising performance, ensuring that systems remain responsive to growing demands.
- Integration with Big Data: AI systems are increasingly integrated with big data analytics, enhancing data analysis capabilities and improving predictive modeling, allowing for more nuanced insights and strategic forecasting.
These trends indicate that financial institutions can leverage AI-based data extraction to optimize workflows, improve customer service, and maintain a competitive advantage. The precision offered by AI-based systems also helps uphold data integrity throughout the extraction process, reinforcing the reliability of financial analyses.
Give Daloopa a Try
Explore how AI-based data extraction overcomes the limitations of manual data entry by providing tailored solutions for investment banking. Dive into the latest innovations and discover how AI-based systems can enhance your financial operations, streamline decision-making, and position your firm for future success. Unlock the full potential of AI in your data extraction processes—learn more with Daloopa today or request a demo and try it out yourself.