The AI revolution has fundamentally transformed the finance industry, especially in investment banking. By integrating AI into financial data management, investment banker analysts can greatly enhance their ability to conduct market research and analysis.
For example, AI-driven tools enable analysts to process and analyze massive volumes of market data quickly, identifying emerging trends such as shifts in consumer behavior or sudden market fluctuations that would take much longer to detect manually.
A practical case involves using AI-powered trading platforms, which analyze historical market data to predict price movements and optimize trade execution. These platforms can anticipate how large orders might impact the market, allowing analysts to execute trades more efficiently and accurately, improving the overall trading strategy.
AI-driven models also enhance predictive capabilities by evaluating risk factors across global markets, allowing analysts to assess the potential impact of geopolitical events or economic changes on their portfolios and enabling them to react to market shifts in near real time.
Moreover, AI’s role in finance extends beyond trading to areas such as customer service, credit scoring, and risk management. AI-powered market research tools allow analysts to process large datasets quickly, yielding deeper insights into market conditions and client behaviors.
Key Takeaways
- AI tools significantly enhance financial data analysis and decision-making in investment banking.
- AI-powered trading platforms improve efficiency and predictive accuracy by analyzing market trends and optimizing trade execution.
- AI-driven market research tools provide deeper insights into market trends and client behaviors, leading to more informed decisions.
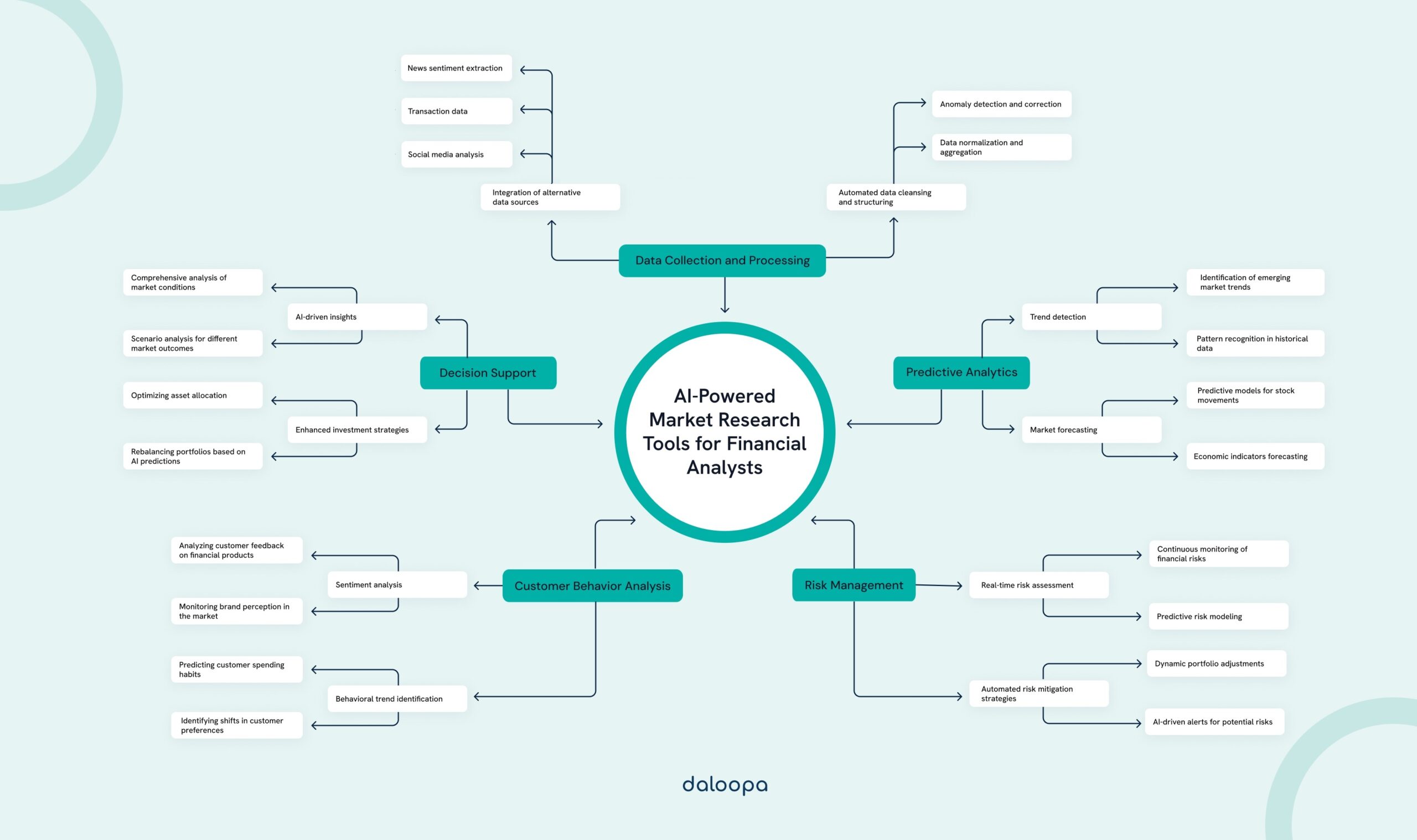
The Evolution of AI in Financial Services
AI has reshaped the financial services industry, particularly in investment banking, by refining decision-making processes, boosting efficiency, and driving innovation.
From the Basics to AI-Powered Solutions
Initially, investment banker analysts relied on basic computational tools to process financial data. Over time, the emergence of machine learning and AI introduced sophisticated algorithms capable of performing predictive analysis. Today, AI enables analysts to automate decision-making with near real-time data processing. For example, AI-driven tools streamline client interactions by providing rapid responses and insights, while predictive maintenance ensures critical systems remain operational, reducing downtime in trading platforms.
AI-powered credit scoring models have evolved to incorporate alternative data sources, refining lending decisions with greater precision. For investment banker analysts, this means access to more nuanced data points that can influence credit risk assessments and portfolio management strategies, resulting in more informed and accurate financial decisions.
Impact on Global GDP and Finance Sector Innovation
The integration of AI into financial services significantly contributes to global GDP by automating complex tasks, reducing operational costs, and enhancing overall productivity. Investment banker analysts utilize it for a more streamlined workflow, allowing them to allocate more time to high-value activities such as strategic decision-making and providing in-depth client advisory services.
Beyond these immediate benefits, AI is also driving substantial innovation within the finance sector, leading to the creation of new financial products and services that are reshaping the industry.
AI-Driven Innovation in Financial Products and Services
AI’s impact on the finance sector extends far beyond improving existing processes, becoming a catalyst for innovation, leading to the development of entirely new financial products and services.
For instance, AI has enabled the rise of Robo-Advisors, which provide automated, algorithm-driven financial planning services with little to no human supervision, using AI to assess an individual’s financial situation and goals and offering personalized investment strategies.
The adoption of Robo-Advisors has made investment management more accessible to a broader audience, particularly those who may not have had access to traditional financial advisory services due to cost barriers.
Another example of AI-driven innovation involves the emergence of smart contracts in the blockchain ecosystem. Smart contracts are self-executing contracts where the terms are directly written into code.
AI enhances these smart contracts by enabling more sophisticated decision-making processes based on real-time data inputs. For investment banker analysts, smart contracts offer new avenues for structuring complex financial products, such as derivatives or syndicated loans, with increased efficiency and reduced counterparty risk.
AI is also at the forefront of personalized financial services. By leveraging machine learning algorithms and big data analytics, financial institutions can offer highly customized financial products tailored to individual customer needs.
For example, some banks are using AI to develop dynamic pricing models for loans and mortgages, where interest rates are adjusted in real-time based on the borrower’s creditworthiness, market conditions, and even social media behavior, enhancing customer satisfaction and optimizing risk management for financial institutions.
Enhancing Data Governance and Security
Investment banker analysts grow increasingly reliant on AI to enhance data governance to ensure that data gets managed securely, efficiently, and in compliance with regulatory standards.
AI tools automate data classification, and AI-driven analytics enable firms to detect and respond to potential data breaches more swiftly, thereby reducing the risk of costly data leaks and ensuring compliance with regulations like the General Data Protection Regulation (GDPR) in Europe or the California Consumer Privacy Act (CCPA) in the United States.
AI also improves the accuracy and efficiency of compliance reporting. By automating the collection and analysis of data across various sources, AI systems can generate reports that meet regulatory requirements with minimal human intervention, freeing up analysts to focus on more strategic tasks.
For instance, AI-powered compliance tools can analyze transactions in real time to detect and flag suspicious activity, ensuring that financial institutions adhere to the Anti-Money Laundering Act (AMLA).
Broader Economic Impact and Sustained Growth
The broader economic implications of AI in finance are profound. As AI automates routine tasks and enhances decision-making capabilities, financial institutions can operate more efficiently, leading to cost savings and improved profitability, contributing to overall economic growth by enabling the finance sector to support more dynamic and innovative economic activities.
Moreover, the adoption of AI technologies in finance contributes to the creation of new markets and revenue streams. For example, the development of AI-driven trading platforms opened up new opportunities for algorithmic trading strategies, which can execute trades at speeds and volumes far beyond human capability, increasing market liquidity and creating new jobs in areas like AI model development, data science, and cybersecurity.
Core Applications of AI in Finance
AI has become a cornerstone of investment banking by enhancing risk management, improving predictive analytics for financial forecasting, and strengthening fraud detection and cybersecurity measures. It provides investment banker analysts with sophisticated tools to make data-driven decisions with greater accuracy and efficiency.
Enhancing Risk Management and Credit Scoring
AI-driven solutions have revolutionized risk management by automating the analysis of financial data. Machine learning algorithms can sift through vast datasets to identify patterns and forecast potential risks, allowing analysts to make proactive decisions and effectively mitigate those risks.
For instance, AI can predict market volatility or assess the financial health of potential investment targets, enabling analysts to craft more resilient investment strategies.
In the realm of credit scoring, AI models assess non-traditional data sources, such as transaction history and behavioral patterns, to improve the accuracy of creditworthiness evaluations.
Predictive Analytics in Financial Forecasting
Predictive analytics, driven by advanced AI algorithms, has become a cornerstone in financial forecasting, leveraging techniques such as time series analysis, neural networks, and regression models to process historical financial data and identify patterns that help forecast future market movements and economic conditions, providing a significant advantage for investment banker analysts by enabling more precise and actionable predictions.
One widely used algorithm in financial forecasting is the Long Short-Term Memory (LSTM) neural network, a type of recurrent neural network (RNN) that excels at processing sequences of data. LSTM models are particularly effective in predicting stock prices, where they analyze time series data to capture long-term dependencies and trends.
For instance, top-tier financial institutions have successfully implemented LSTM models to forecast stock market movements, improving prediction accuracy by up to 30% compared to traditional methods.
Another critical AI model in predictive analytics is the Random Forest algorithm, which is often used for macroeconomic forecasting and combines multiple decision trees to improve predictive accuracy and handle large, complex datasets.
For example, investment banks have employed Random Forest models to assess the impact of various economic indicators on financial markets, helping analysts refine their investment strategies and better anticipate market shifts.
In practice, AI-driven forecasting models are invaluable for tasks like budget planning, investment analysis, and strategic decision-making. A case in point involves a leading financial firm using AI to predict the impact of monetary policy changes on bond markets.
By analyzing historical data and current economic conditions, the AI model provided analysts with forecasts that were 20% more accurate than those generated through traditional econometric models, allowing the firm to optimize its bond portfolio and improve overall returns.
AI-Driven Fraud Detection and Cybersecurity
AI enhances fraud detection and cybersecurity within the financial sector. Machine learning algorithms such as anomaly detection models and deep learning techniques help identify suspicious activities in real time by analyzing transaction patterns and flagging anomalies that could indicate fraudulent behavior.
One example of a widely implemented algorithm is the Isolation Forest, specifically designed for anomaly detection, isolating observations by randomly selecting a feature and then selecting a split value between the maximum and minimum values of the selected feature. Transactions that require fewer random splits to be isolated are deemed anomalies.
Top financial institutions have integrated Isolation Forest algorithms into their fraud detection systems, reducing false positives by 50% and enabling quicker identification of fraudulent transactions.
Deep learning models, particularly Convolutional Neural Networks (CNNs), are also employed in fraud detection. CNNs are typically associated with image processing adapted to detect patterns in transactional data.
By analyzing vast datasets, CNNs can detect subtle anomalies that might go unnoticed by traditional models. Leading banks have used CNN-based systems to enhance their fraud detection capabilities, reducing fraud-related losses by millions of dollars annually.
In cybersecurity, AI-powered systems continuously monitor network activities to detect potential cyber threats. Algorithms such as Deep Belief Networks (DBNs) are employed to analyze network traffic and identify patterns associated with cyber attacks, adapting and evolving by learning from new data and staying ahead of increasingly sophisticated cyber threats.
Thanks to DBNs, Investment banker analysts can safeguard sensitive financial information and ensure compliance with regulatory standards. Successful implementations of AI-driven cybersecurity systems in financial institutions have led to a 40% reduction in breach attempts, significantly enhancing client trust and the overall security posture of these organizations.
AI-Enhanced Customer Experiences in Finance
Advanced AI technologies are revolutionizing how financial institutions interact with clients. Key areas of impact include personalized services through chatbots and virtual assistants, as well as improved customer engagement and service delivery.
Personalization Through AI Chatbots and Virtual Assistants
AI chatbots and virtual assistants personalize client interactions by analyzing data to provide tailored financial recommendations. For instance, a chatbot can assess a client’s spending patterns and suggest relevant financial products, which helps analysts offer more precise advice.
Additionally, these tools offer 24/7 support, ensuring clients receive prompt responses to their inquiries, enhancing satisfaction and loyalty, allowing analysts to focus on more complex client needs, and improving efficiency.
Improving Customer Engagement and Service
AI-driven tools boost customer engagement by predicting client needs and proactively offering solutions. For financial analysts, this means better-prepared conversations with clients, as AI can identify potential areas of interest or concern. Personalized notifications and campaigns further enhance engagement by aligning messages with client behavior, increasing the likelihood of positive outcomes.
Moreover, AI systems monitor client feedback, allowing analysts to continuously refine their approach and improve service quality, leading to higher client satisfaction and retention.
Operational Efficiency and Process Automation
Operational efficiency and process automation play crucial roles in improving the productivity and accuracy of financial data management. They enable firms to automate routine tasks and enhance decision-making through advanced cognitive technologies.
Optimizing Workflows with Robotic Process Automation
Robotic Process Automation (RPA) is instrumental in automating repetitive, rule-based tasks that often consume substantial time and resources. In financial institutions, RPA has been used to streamline workflows such as data entry, transaction processing, and report generation.
RPA is also highly effective in compliance and regulatory reporting, where accuracy and timeliness are critical. By automating these processes, financial institutions have seen a reduction in manual input errors by up to 90%, according to industry reports, minimizing the risk of non-compliance and enhancing the reliability of the data used for decision-making. As a result, analysts can trust the data they work with, leading to more confident and accurate financial strategies.
Boosting Efficiency through Cognitive Automation
Cognitive automation extends the capabilities of RPA by incorporating artificial intelligence elements such as machine learning and natural language processing, allowing financial institutions to automate more complex tasks that require decision-making and analytical capabilities. For instance, cognitive automation can analyze unstructured data, such as market news or social media sentiment, to provide insights crucial for financial analysis and strategic planning.
Overall, the integration of RPA and cognitive automation not only boosts operational efficiency but also empowers financial analysts to be more agile in their decision-making. This agility is critical in responding quickly to market changes, ultimately leading to better financial performance and a competitive edge in the industry.
Regulatory Compliance and Governance
In dealing with financial data management, ensuring regulatory compliance and effective governance frameworks includes the vital interplay between AI integration and human oversight to uphold standards.
Ensuring Compliance with AI
AI technologies play a crucial role in regulatory compliance by automating complex reporting processes and ensuring accuracy. These systems can analyze vast datasets and identify patterns that might elude human analysts. For example, AI-powered regulatory reporting tools can streamline compliance with financial regulations by providing real-time data analysis and reducing manual errors.
Financial institutions must also adhere to evolving regulatory standards to avoid penalties. AI systems can be programmed to incorporate changes in regulations, ensuring that institutions remain compliant with the most current laws. Additionally, the use of AI promotes transparency in data management, which simplifies auditing processes and helps maintain trust with regulatory bodies.
The Role of Human Oversight in AI Governance
Despite the capabilities of AI, human oversight remains essential to govern these systems effectively. People must interpret AI-driven insights, make strategic decisions, and ensure they meet ethical considerations. We cannot rely solely on AI for compliance and governance because the nuanced understanding of regulatory requirements often requires human judgment.
Establishing an ethical framework for AI governance includes setting boundaries for AI decision-making, ensuring accountability, and regularly reviewing AI algorithms to prevent biases.
Advanced AI Technologies Shaping Finance
AI technologies enable detailed market analysis, enhanced decision-making, and sophisticated algorithmic trading strategies.
Leveraging Machine Learning and Deep Learning
Machine Learning and Deep Learning are at the forefront of financial innovation. Machine Learning enables predictive analytics by identifying patterns in historical market data, enhancing the accuracy of financial forecasts.
Deep Learning, a subset of Machine Learning, utilizes neural networks to process large datasets, allowing for deeper insights into complex financial trends. Financial institutions use these technologies to optimize portfolio management, credit scoring, and risk assessment, ensuring data-driven decision-making processes.
Natural Language Processing for Enhanced Analytics
Natural Language Processing (NLP) extracts valuable insights from unstructured data like news articles, financial reports, and social media. Natural Language Processing technologies analyze sentiment and detect emerging market trends, providing analysts with real-time information and enhancing market research by integrating qualitative data into traditional quantitative analyses, resulting in a comprehensive understanding of market movements. AI-powered tools can automatically generate summaries of lengthy financial documents, streamlining data processing tasks.
Challenges and Considerations in AI Adoption
While leveraging AI in financial data management presents transformative opportunities, the adoption of these technologies involves several complexities. We must address the ethical considerations, ensure data privacy, and invest in upskilling our talent pool.
Data Privacy and Security Issues
AI-powered tools rely on massive volumes of data, often containing sensitive financial and personal information, raising significant concerns regarding data privacy and security. Unauthorized access or data breaches can lead to severe consequences, including financial loss and reputational damage.
To mitigate these risks, we should adopt advanced encryption techniques and multi-factor authentication for data access. Establishing stringent data governance policies ensures compliance with regulatory standards like GDPR and CCPA. Regular security audits and employing AI-based cybersecurity tools can further enhance the protection of sensitive information.
Addressing Bias and Ethics in AI Systems
AI systems in finance carry significant potential for both positive impact and unintended consequences, particularly concerning bias and ethics. AI models are highly dependent on the data they are trained on and the algorithms that process this data.
If the input data contains biases—such as historical discrimination in lending practices—AI systems may perpetuate or even exacerbate these biases, leading to unethical outcomes like discriminatory credit scoring or lending decisions.
The Role of Data in Bias
Bias in AI systems often stems from biased or unrepresentative data. For instance, if an AI model used for credit scoring is trained on data where certain demographic groups have historically been underserved or denied credit, the AI could replicate this bias, resulting in unfair treatment of those groups.
Regulatory Guidelines and Upcoming Regulations
Regulatory bodies are increasingly recognizing the importance of addressing bias and ensuring ethical AI use in finance. For example, the European Union’s proposed Artificial Intelligence Act (AI Act) specifically targets high-risk AI applications, including those in financial services.
The AI Act mandates that AI systems in finance must adhere to strict requirements for transparency, accountability, and bias mitigation. Financial institutions will need to perform regular audits of their AI systems, ensure that the data used is representative, and document their AI processes to comply with these regulations.
In the United States, the Consumer Financial Protection Bureau (CFPB) has issued guidance on the use of AI in credit decisions, emphasizing the need for transparency and fairness.
The CFPB’s regulations, under the Equal Credit Opportunity Act (ECOA), require financial institutions to explain adverse credit decisions to consumers, which has implications for AI models that must be interpretable and free from discriminatory bias.
Moreover, the Algorithmic Accountability Act (AAA) proposed in the U.S. Congress would require companies, including those in finance, to conduct impact assessments on their automated systems, ensuring that AI does not reinforce existing inequalities. This act, if passed, would mandate organizations to evaluate the potential bias in their AI systems and take steps to mitigate it.
Ethical Frameworks and Auditing
To address bias, financial institutions must implement robust frameworks for regular auditing and updating their AI models and datasets, involving technical measures, such as using de-biasing algorithms or re-sampling datasets to ensure they are representative, and organizational practices, like establishing ethical review boards.
Transparent AI algorithms are crucial for maintaining fairness and accountability, providing clear insights into their decision-making processes, and allowing for external audits and regulatory compliance.
For example, Explainable Artificial intelligence (XAI) techniques can be employed to ensure that decisions made by AI systems can be traced back to specific data inputs and logic, making it easier to detect and correct biased outcomes.
Ethical Guidelines in AI Usage
Financial institutions are increasingly adopting ethical guidelines to govern the use of AI, ensuring that these technologies do not perpetuate or amplify existing inequalities. These guidelines typically include principles of fairness, transparency, and accountability.
For example, organizations may implement policies that require AI systems to undergo rigorous testing for bias before deployment and mandate regular reviews of AI-driven decisions, particularly in sensitive areas like lending and investment.
Furthermore, industry groups like the Global Financial Markets Association (GFMA) and the Institute of International Finance (IIF) are developing best practices and standards for ethical AI use in finance. These standards often align with broader international frameworks, such as the OECD AI Principles, which advocate for human-centric AI that respects human rights and promotes fairness.
The Future of AI in Financial Data Analysis
As AI technology rapidly advances, we are on the cusp of a significant transformation in how financial data is analyzed. Emerging trends in AI are poised to disrupt traditional market research practices, offering unprecedented insights and capabilities, enhancing our ability to predict market trends, optimizing portfolio management, and redefining the tools and methods used by financial analysts.
Predicting Market Trends with AI
The future of market trend analysis lies in AI’s ability to process and interpret vast, complex datasets with increasing speed and accuracy. Advanced machine learning models are evolving to identify patterns and anomalies that traditional methods might overlook.
One of the most promising trends is the use of generative AI in financial modeling. Generative AI can create new data points or simulations based on existing data, offering fresh perspectives on market scenarios and revolutionizing how analysts conduct stress tests and scenario planning, allowing them to explore a broader range of potential market conditions and outcomes.
AI in Portfolio Management and Investment Strategy: A Glimpse Ahead
In portfolio management, AI’s role is set to expand significantly. Future AI algorithms will not only optimize asset allocation and manage risks more efficiently but will also incorporate reinforcement learning—a type of machine learning where models learn to make decisions by evaluating the outcomes of previous actions. This approach will enable AI to continuously improve its investment strategies by learning from real-world market feedback.
AI-powered robo-advisors will become even more sophisticated, offering hyper-personalized investment strategies that adjust dynamically as an investor’s financial situation or market conditions change. These systems could evolve to include AI-driven behavioral finance models that take into account not just financial data but also psychological factors that influence investor decisions, offering more holistic and tailored advice.
Furthermore, AI will increasingly automate routine portfolio management tasks, such as rebalancing and tax-loss harvesting. The next wave of AI tools will likely incorporate predictive analytics to forecast not just market trends but also potential disruptions—such as economic downturns or geopolitical events—enabling more proactive adjustments to investment strategies.
AI’s role in predictive maintenance within portfolio management will also evolve. Instead of merely reacting to financial issues as they arise, future AI systems will predict potential risks before they materialize, allowing for preemptive measures that mitigate losses and capitalize on emerging opportunities. This will lead to investment strategies that are not only more resilient but also more adaptive to a rapidly changing financial landscape.
Are You Harnessing the Full Power of AI in Your Market Research?
As AI continues to transform financial data analysis, the tools available to analysts will become increasingly powerful and indispensable. Traditional market research methods, reliant on manual data processing and analysis, will be eclipsed by AI-driven solutions that offer real-time insights and unparalleled predictive accuracy.
In the near future, financial institutions that embrace these cutting-edge AI tools will gain a significant competitive edge. Whether it’s through enhanced sentiment analysis, dynamic portfolio management, or innovative use of generative AI, the next generation of financial products and services will be defined by the capabilities of AI.
Don’t miss out on the competitive edge that AI can offer. Create your free Daloopa account today to harness real-time data analysis and automated trend detection, directly addressing the challenges of staying ahead in a fast-moving market. With Daloopa’s advanced tools, you can streamline your research process, gain deeper insights, and make data-driven decisions with confidence. Revolutionize your market research and decision-making process now.