In today’s highly competitive investment landscape, hedge funds are turning to alternative data to uncover hidden insights and outpace their rivals. Alternative data refers to non-traditional sources such as social media sentiment, satellite imagery, and real-time e-commerce trends, offering a unique lens into market conditions and often revealing shifts before traditional data providers catch on. For instance, monitoring retail foot traffic through geolocation data provides hedge funds with valuable insights into consumer behavior, allowing them to adjust portfolios swiftly in response to emerging trends.
Beyond the technical ability to deliver high-quality, timely data, hedge funds must also navigate the legal and ethical complexities associated with these sources. A well-vetted provider significantly enhances a fund’s ability to generate alpha, helping them anticipate market changes and capitalize on profitable opportunities ahead of their competitors.
Key Takeaways
- Enhanced Predictive Accuracy: Integrating alternative data into hedge fund strategies has been shown to improve predictive accuracy by up to 25% in certain trading models, particularly those focused on macroeconomic trends or consumer behavior. Analysts should consider testing these data sets alongside traditional models to assess their impact on forecasting performance.
- Diverse and Innovative Data Sources: Alternative data extends beyond conventional financial metrics, drawing from sources such as geospatial data, web traffic, and social sentiment analysis. By combining multiple data types, analysts can uncover patterns not visible through traditional methods, enhancing decision-making in volatile markets.
- Navigating Legal and Ethical Complexities: The use of alternative data requires a deep understanding of both legal regulations and ethical guidelines. Analysts should work closely with compliance teams to ensure data sourcing adheres to privacy laws and avoid using data that could expose the fund to regulatory risks. Proactively addressing these concerns safeguards long-term investment strategies.
The Evolution of Data in Hedge Fund Management
The transformation of data usage in hedge fund management has been profound, transitioning from reliance on traditional data sources to the integration of alternative data, AI, and machine learning.
From Traditional to Alternative Data
Historically, hedge funds primarily relied on traditional data sources like financial statements, stock price movements, and economic indicators. These conventional metrics formed the backbone of fundamental and quantitative analysis. However, the rise of digitalization in the early 2000s began expanding the types of data hedge funds could access. A pivotal shift occurred in the mid-2010s when alternative data—information derived from non-traditional sources—gained widespread traction.
Today, hedge funds regularly incorporate data from social media sentiment, satellite imagery, geolocation, and transaction data into their models. According to a 2022 report by Preqin, 78% of hedge funds now integrate some form of alternative data into their investment strategies, allowing managers to uncover patterns that traditional data sets cannot reveal, improving predictive accuracy and market foresight. For instance, satellite imagery used to track retail parking lot activity can give funds real-time insights into consumer demand well ahead of earnings reports.
Role of AI and Machine Learning
The introduction of AI and machine learning in the late 2000s has further accelerated the data revolution in hedge funds. These technologies enable funds to process vast quantities of both traditional and alternative data with unprecedented speed and precision. Advanced machine learning models can identify intricate relationships within datasets, leading to more accurate predictions and a deeper understanding of market dynamics.
For example, in 2021, a hedge fund that integrated machine learning algorithms into its trading models reported a 30% improvement in forecast accuracy for commodity prices compared to traditional analysis methods. As highlighted in a 2023 study by Deloitte, the ability of AI to continuously learn from new data sets provides hedge funds with a dynamic edge, allowing for responsive and adaptive investment strategies.
The integration of AI also drives advancements in risk management. By utilizing AI-powered tools, hedge funds can conduct real-time risk assessments and adjust portfolios swiftly in response to market volatility. The role of these emerging technologies in hedge funds underscores the importance of continued innovation, as discussed in industry analyses like the CFA Institute’s report on the future of alternative investments.
Types of Alternative Data Used by Hedge Funds: Detailed Insights and Challenges
Alternative data plays an increasingly critical role for hedge funds, offering unique insights that traditional sources often miss. From consumer spending patterns to expert networks, hedge funds leverage various forms of alternative data to refine investment strategies. However, utilizing this data effectively requires overcoming challenges related to data quality, integration, and reliability. Below, we explore several key types of alternative data, their successful applications, challenges, and mitigation strategies.
1. Consumer Spending Data
Consumer spending data provides real-time insights into purchasing behavior, allowing hedge funds to forecast market trends and economic activity. A prime example occurred in 2020 when hedge funds used aggregated credit card transaction data to anticipate shifts in consumer spending during the pandemic. Funds that tracked increased spending on e-commerce platforms like Amazon successfully capitalized on the stock’s surge, generating returns above industry averages. A 2021 study by Refinitiv found that hedge funds leveraging consumer spending data saw a 10% improvement in quarterly stock prediction accuracy.
Challenges:
- Data Fragmentation: Consumer spending data comes from multiple sources (credit cards, point-of-sale, mobile payments), making it difficult to create a unified dataset.
- Mitigation: Hedge funds partner with specialized data aggregators that provide pre-processed, anonymized transaction data, ensuring higher accuracy and reducing the need for extensive data cleaning.
2. Social Media Sentiment and Online Reviews
Social media sentiment data offers hedge funds a real-time pulse on public perception and market sentiment. In 2021, a well-known hedge fund used sentiment analysis on Twitter and Reddit to monitor the chatter around meme stocks like GameStop. By interpreting positive sentiment spikes, the fund made a timely long bet on the stock, securing substantial profits during the short squeeze. According to a 2022 report by PwC, hedge funds using social media data saw a 15% increase in the accuracy of short-term stock price forecasts.
Challenges:
- Data Noise: Social media data is often noisy, with irrelevant or misleading information skewing sentiment analysis.
- Mitigation: Funds employ natural language processing (NLP) tools to filter out non-relevant content and focus on actionable insights. Cross-referencing sentiment data with market movements helps validate its reliability.
3. Web Crawled Data
Web-crawled data involves the automated extraction of publicly available information from websites, such as job postings, regulatory filings, and product announcements, aiding in monitoring corporate activity and detecting market-moving information. For instance, in 2019, a hedge fund tracked job postings related to artificial intelligence on LinkedIn to identify a technology company’s pivot toward AI, leading to a timely investment ahead of public announcements, yielding significant gains when the stock surged following the company’s official AI initiative announcement.
Challenges:
- Data Quality: Web crawlers can capture outdated or irrelevant information, making it difficult to ensure data accuracy.
- Mitigation: Hedge funds rely on machine learning algorithms to validate and clean crawled data. They also monitor specific keywords and real-time news sources to ensure relevance.
4. Expert Networks
Expert networks provide hedge funds with access to industry professionals, offering deep, qualitative insights into specific markets or sectors. For example, hedge funds consulting experts from the pharmaceutical industry during the COVID-19 vaccine development race were able to predict which companies were ahead in clinical trials, leading to early, profitable investments in biotech stocks like Moderna and Pfizer before their stock prices surged. According to a 2022 survey by Integrity Research, funds using expert networks reported a 20% improvement in their ability to identify emerging market trends.
Challenges:
- Bias and Reliability: Expert opinions can sometimes be subjective or overly optimistic, leading to biased investment decisions.
- Mitigation: Funds cross-check expert insights with other quantitative data sources, ensuring that opinions are supported by market data and trends.
5. Business Performance Metrics
Hedge funds use business performance metrics such as sales growth, supply chain efficiency, and inventory levels to evaluate a company’s operational health. In 2022, a hedge fund monitored supply chain disruptions at a major electronics manufacturer, noticing that increased inventory turnover in raw materials indicated a recovery in production. This led to an early long position on the stock, which later surged as the company reported strong earnings. Research from McKinsey in 2023 shows that hedge funds using operational metrics have improved earnings prediction accuracy by 18%.
Challenges:
- Data Timeliness: Accessing real-time business metrics can be difficult, as many of these insights are reported on a delayed basis.
- Mitigation: Hedge funds often integrate real-time alternative data sources like geolocation and web traffic to complement traditional business metrics, providing a fuller picture of a company’s performance.
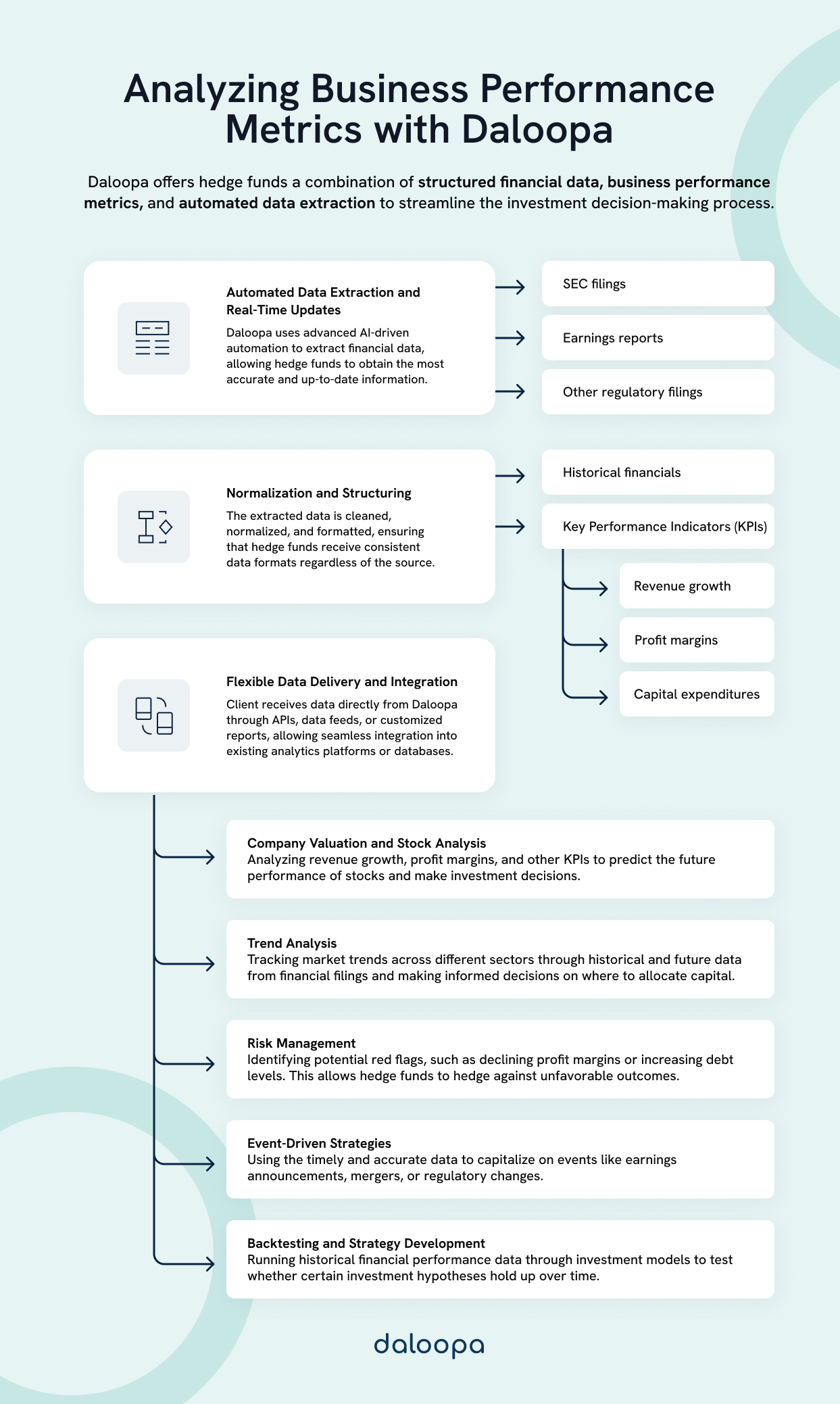
Comparative Analysis of Leading Alternative Data Providers for Hedge Funds
In today’s competitive landscape, hedge funds increasingly rely on alternative data to generate alpha and improve decision-making.
Comparative Table of Leading Providers
Provider | Key Data Types | Strengths | Weaknesses | Integration & Pricing | Case Study/Use |
Daloopa | Business performance metrics | Automated financial data extraction | Limited to structured financial data | API, Excel, Transparent pricing | Hedge funds have cut financial model creation time by 70% using Daloopa’s automated financial filings. |
Quandl | Satellite imagery, financial & alternative | Easy API integration; wide dataset coverage | Can overwhelm with excess data | API; Subscription-based | A hedge fund using Quandl’s satellite imagery predicted retail performance, resulting in 15% portfolio growth. |
YipitData | Consumer transaction data | Strong predictive analytics for consumer trends | Lacks comprehensive coverage outside of retail | API; Premium pricing | YipitData predicted holiday spending surges in e-commerce, driving a 12% increase in a client’s ROI. |
Thinknum | Web-sourced data (job postings, social media) | Real-time updates, visualizations | Accuracy may vary with unverified web sources | Web-based, Tiered pricing | A fund used Thinknum’s job posting data to anticipate a tech company’s expansion, leading to early investment gains. |
RS Metrics | Satellite imagery, ESG data | ESG analytics for socially responsible funds | High cost of satellite imagery | Custom integration; High pricing | RS Metrics helped a hedge fund identify underperforming retail locations, improving short-selling strategies. |
Second Measure | Consumer transaction data | Real-time transaction tracking | Limited to specific sectors (e.g., retail) | API; Subscription-based | A client used Second Measure to track a decline in restaurant chain sales, enabling timely stock divestment. |
M Science | Social media, e-commerce data | Customizable research reports | Processing of unrefined data can be slow | API, Excel, Flexible pricing | M Science’s social media sentiment analysis identified growth potential in an e-commerce company, leading to a profitable position. |
Eagle Alpha | Broad alternative data, macroeconomic data | Data sourcing and expert consultation | Requires significant cleaning and prep | API; Enterprise pricing | Eagle Alpha provided cross-sectional industry data, improving a hedge fund’s sectoral analysis accuracy. |
Earnest Analytics | Consumer transaction data | Reliable and transparent data | Limited to consumer sectors | Web-based; Subscription | Earnest’s transaction data enabled a hedge fund to accurately predict retail trends during holiday seasons. |
Orbital Insight | Geospatial data, satellite imagery | Global economic visibility | High learning curve for interpretation | API; Enterprise pricing | Orbital Insight helped a hedge fund predict commodity price movements by monitoring global oil reserves via satellite imagery. |
In-Depth Provider Analysis
1. Daloopa
- Overview: Daloopa specializes in automating the collection and structuring of financial data, particularly from company filings, earnings reports, and market data.
- Strengths: Daloopa’s AI-driven platform reduces time spent on financial modeling by automating data extraction and analysis. Hedge funds report a 70% reduction in time spent gathering and structuring data for analysis.
- Challenges: Daloopa’s focus on structured financial data may limit its utility for funds looking to incorporate more diverse alternative datasets.
- Use Case: A hedge fund used Daloopa’s automated financial model updates to outperform peers during earnings season by reacting faster to corporate filings.
2. Quandl
- Overview: Quandl offers a wide array of datasets, ranging from traditional financial data to satellite imagery and alternative economic data.
- Strengths: Its ease of integration with Python, Excel, and R makes it a versatile choice for hedge funds. The breadth of datasets allows for unique insights across industries.
- Challenges: The sheer volume of data can be overwhelming, and not all datasets are equally useful, which can complicate decision-making.
- Use Case: A hedge fund used Quandl’s satellite imagery to analyze retail parking lot traffic, accurately predicting Q4 earnings for a major retailer and driving significant portfolio growth.
3. YipitData
- Overview: YipitData excels at delivering aggregated consumer transaction data, helping funds analyze spending patterns to predict market trends.
- Strengths: Their deep focus on consumer sectors makes them particularly valuable for hedge funds targeting retail and e-commerce stocks. Predictive analytics allow for forward-looking insights.
- Challenges: YipitData’s strengths in consumer spending data are not as applicable to funds focusing on sectors like industrials or technology.
- Use Case: A hedge fund used YipitData’s insights to capitalize on a surge in online retail spending during the holiday season, resulting in a 12% increase in returns.
4. Thinknum
- Overview: Thinknum specializes in web-sourced alternative datasets, including data from job listings, social media, and company websites.
- Strengths: The platform’s real-time data and visualizations allow hedge funds to monitor corporate growth trends and operational health efficiently.
- Challenges: Web-sourced data can be prone to inaccuracies or misinterpretations, especially when derived from unverified online sources.
- Use Case: A hedge fund identified a surge in job postings from a leading tech firm using Thinknum, positioning itself early before the company announced a major expansion, leading to a lucrative investment.
5. RS Metrics
- Overview: RS Metrics provides satellite-based geospatial data with a focus on asset-level performance and ESG insights.
- Strengths: The firm’s satellite imagery allows hedge funds to gain visibility into physical assets and monitor environmental and social metrics.
- Challenges: Satellite images cost quite a bit and may require specialized knowledge to interpret effectively.
- Use Case: RS Metrics helped a hedge fund analyze foot traffic at a large retail chain, identifying a downturn that led to a successful short-selling strategy.
6. Second Measure
- Overview: Second Measure specializes in analyzing consumer transaction data, offering insights into spending habits and brand loyalty. The platform’s real-time transaction analytics are particularly useful for tracking trends in consumer discretionary sectors, such as retail and restaurants.
- Strengths: Real-time transaction data allows hedge funds to assess company performance before releasing official earnings reports. Second Measure’s data is highly actionable, providing forward-looking insights into consumer behavior trends.
- Challenges: The platform is limited to sectors that rely heavily on consumer transactions, such as retail and dining, which may not suit funds focused on industrials or other non-consumer sectors.
- Use Case: A hedge fund used Second Measure’s transaction data to detect a decline in consumer spending at a major restaurant chain before earnings reports were released. The early insight allowed the fund to adjust its position, avoiding significant losses.
7. M Science
- Overview: M Science delivers actionable insights by analyzing various alternative datasets, including social media mentions, consumer transaction data, and e-commerce statistics. Their research combines alternative and traditional datasets to give hedge funds a more comprehensive view of company performance and emerging trends.
- Strengths: The platform offers customizable research options, allowing hedge funds to tailor their analysis to specific sectors or investment strategies. M Science’s proprietary methods extract valuable insights from unstructured data sources, improving the accuracy of predictive models.
- Challenges: Processing unrefined alternative datasets can sometimes be slow, and the platform’s depth may require a steep learning curve for analysts unfamiliar with specific industries or data types.
- Use Case: A hedge fund used M Science’s analysis of e-commerce sales data and social media mentions to anticipate a surge in a leading tech company’s stock price, resulting in significant gains after the company exceeded earnings expectations.
8. Eagle Alpha
- Overview: Eagle Alpha offers a comprehensive suite of alternative data services, including data sourcing, cleaning, and analysis, across various industries and macroeconomic sectors. Their platform provides curated data that helps hedge funds discover alpha-generating opportunities, particularly for macroeconomic and industry-level analysis.
- Strengths: Eagle Alpha’s platform boasts high customization abilities, allowing hedge funds to access data based on specific investment interests. The platform’s expert consultation service enables funds to optimize their use of alternative data by working directly with data specialists.
- Challenges: While Eagle Alpha’s comprehensive offerings are a strength, the vast amount of data may require significant cleaning and preparation before use, which can be time-consuming.
- Use Case: A hedge fund used Eagle Alpha’s cross-industry data to enhance its macroeconomic analysis, identifying trends in energy consumption that led to early positioning in emerging markets and driving portfolio performance.
9. Earnest Analytics
- Overview: Earnest Analytics focuses on providing detailed insights into consumer behavior through transaction data. Their data benefits sectors like retail, restaurants, and technology, where consumer spending patterns are key indicators of company performance.
- Strengths: Earnest Analytics places a strong emphasis on data transparency and quality, sourcing data from reputable origins, making it easier for hedge funds to trust the reliability of the insights and integrate them into their investment models.
- Challenges: The platform’s focus on consumer-driven sectors means it has limited application for funds invested in industrial or infrastructure-heavy markets.
- Use Case: Earnest Analytics enabled a hedge fund to monitor shifts in consumer spending patterns leading up to a major holiday season, allowing the fund to predict which retail companies would outperform and adjust their positions accordingly.
10. Orbital Insight
- Overview: Orbital Insight leverages satellite imagery and geospatial analytics to track global economic indicators, providing strategic insights into industries ranging from agriculture to construction. By using AI to interpret satellite images, Orbital Insight delivers timely intelligence on global market conditions, supply chains, and asset-level performance.
- Strengths: Orbital Insight’s geospatial data offers unique visibility into macroeconomic conditions and asset-level details that are difficult to obtain through traditional data sources, allowing hedge funds to anticipate global market shifts more accurately.
- Challenges: Interpreting large volumes of satellite data requires specialized knowledge, which may limit its use to hedge funds with the expertise and resources to fully understand the implications of the data. Additionally, the high cost of satellite imagery services can be a barrier for smaller funds.
- Use Case: Orbital Insight helped a hedge fund accurately predict fluctuations in global oil supply by monitoring the volume of crude oil stored in tankers and storage facilities, allowing the fund to position itself advantageously in the energy markets, significantly boosting its returns during a volatile period.
Regulatory Compliance and Data Quality Challenges
Across all providers, hedge funds must navigate complex regulatory and data quality challenges. Ensuring compliance with data privacy laws like GDPR and CCPA takes high importance when dealing with consumer data. Providers like Earnest Analytics and Second Measure emphasize transparency and data sourcing compliance, helping funds mitigate legal risks.
- Data Quality: Issues such as data fragmentation (as seen with consumer transaction data) or noise (social media sentiment) can complicate analysis. Providers like M Science and YipitData employ advanced cleaning and validation techniques to improve data reliability.
- Mitigation: Hedge funds often supplement alternative data with traditional datasets to verify findings. Working with providers that offer comprehensive compliance support, like Quandl and RS Metrics, can further reduce legal and ethical risks.
By understanding the strengths and limitations of each provider, hedge funds can make more informed decisions on which alternative data provider best suits their specific needs, improving overall performance and risk management.
Ethical and Legal Considerations in Alternative Data Usage
Regulatory bodies like the SEC, the European Union’s GDPR, and California’s CCPA have established stringent guidelines on data usage, privacy, and transparency.
Compliance with Key Regulations
- SEC Guidelines: The U.S. Securities and Exchange Commission (SEC) mandates that hedge funds avoid using material non-public information (MNPI), influencing financial markets unfairly. Hedge funds must ensure that alternative data sources do not contain MNPI. For instance, datasets derived from private corporate communications or confidential business operations would fall under this prohibition.
Compliance Strategy: Implement robust policies to distinguish public data from non-public sources. Hedge funds should deploy compliance officers who rigorously audit datasets to ensure no MNPI is used. Tools like automated compliance systems can assist in identifying risky data points, while regular staff training ensures employees understand how to avoid potential violations. Regular audits and detailed documentation of data acquisition processes are essential for demonstrating compliance during regulatory reviews. - GDPR (General Data Protection Regulation): Hedge funds dealing with European data subjects must comply with the GDPR, requiring explicit consent to be obtained from individuals before using their personal data, and firms must ensure the legal processing of personal data. The right to be forgotten and data minimization principles are central to GDPR compliance.
Compliance Strategy: Hedge funds must ensure that all data vendors provide documentation confirming GDPR compliance, especially regarding data subjects’ consent. Data privacy impact assessments (DPIAs) should be conducted for all data sources to identify potential risks. Additionally, a data protection officer (DPO) should be appointed to oversee GDPR compliance, and the firm should implement mechanisms to handle requests for data deletion or access from individuals promptly. - CCPA (California Consumer Privacy Act): The CCPA grants California residents the right to know what personal data is collected about them and how it is used. Hedge funds need to ensure they respect opt-out requests and provide clear disclosures about data usage.
Compliance Strategy: Hedge funds should offer user-friendly mechanisms for individuals to opt out of data collection. They should also create detailed privacy policies outlining how consumer data gets collected, used, and shared.
Ethical Data Sourcing and Transparency
Ethically sourcing alternative data means ensuring that the data is acquired legally and with respect for individual privacy rights, involving adhering to regulations and a commitment to transparency and ethical decision-making.
- Vendor Due Diligence: Hedge funds must vet data vendors to ensure their data is collected in an ethical and lawful manner, including verifying that the vendors are compliant with relevant laws such as GDPR, CCPA, and any sector-specific regulations. Hedge funds should also confirm that vendors have obtained the necessary consent from individuals whose data is being sold or analyzed.
Compliance Strategy: Create a comprehensive vendor due diligence checklist that covers data collection methods, privacy policies, and regulatory compliance. Hedge funds should also maintain detailed contracts with data vendors, specifying the responsibilities of both parties in case of a regulatory breach. Furthermore, vendor practices must be regularly reviewed to ensure ongoing compliance with changing regulations. - Transparency with Clients and Regulators: Hedge funds should be transparent with clients and regulators regarding how alternative data is sourced and used. Clear disclosure in client communications, as well as regular reporting to regulatory bodies, helps to build trust and mitigate potential legal exposure.
Compliance Strategy: Develop transparent reporting frameworks that detail the origin of datasets, methods of analysis, and the ethical safeguards in place. Establish clear communication channels with regulators and proactively disclose any compliance concerns or potential breaches, demonstrating a commitment to ethical practices and helping mitigate legal risks by ensuring continuous regulatory alignment.
Avoiding Material Non-Public Information (MNPI)
The increasing reliance on alternative data exposes hedge funds to the heightened risk of inadvertently accessing Material Non-Public Information (MNPI)—private corporate information that, if disclosed, could impact market prices. The SEC’s Regulation Fair Disclosure and rules governing insider trading make it clear that using MNPI for trading decisions can lead to severe legal and regulatory consequences, including fines, reputational damage, and operational disruption. Hedge funds must take proactive measures to mitigate these risks.
Best Practices for Avoiding MNPI
- Thorough Data Vendor Vetting One of the primary risks associated with alternative data is the sourcing of MNPI through third-party vendors. Hedge funds should perform rigorous due diligence on data vendors to ensure that they follow ethical and legal practices in gathering data. Vendors must certify that their data does not include MNPI and complies with privacy regulations like GDPR and CCPA.
Action Steps:- Request Data Collection Processes: Ensure that vendors provide documentation on how data is collected, including details on consent and the legality of its sourcing.
- Contractual Safeguards: Hedge funds should include MNPI-related clauses in their vendor agreements, ensuring that vendors are liable if MNPI is found in their data.
- Third-Party Audits: Consider working with external legal and compliance experts to audit vendors regularly and verify the transparency of their data collection methods.
- Multi-Tiered Compliance Review Implementing a multi-tiered review process is critical to identifying and avoiding MNPI. Automated tools, in conjunction with observance from a person, screen large datasets to detect potential MNPI. Hedge funds should maintain a compliance team tasked with reviewing flagged data points manually, focusing on high-risk areas like corporate communications, undisclosed earnings information, or proprietary financial metrics.
Action Steps:- Automated Screening: Use AI-driven tools that can flag potential MNPI in datasets based on specific risk factors, such as keywords related to corporate strategies or private deals.
- Manual Review by Compliance Teams: After automated flags, a compliance team should manually inspect any questionable data to ensure no non-public information is being used.
- Internal Audits: Conduct regular internal audits to assess the efficacy of both automated and manual processes and adjust screening protocols based on evolving legal standards.
- Employee Training and Awareness Programs Employees are often the first line of defense in preventing the use of MNPI. Therefore, regular compliance training programs are vital for educating staff on recognizing potential MNPI and understanding the legal consequences of its misuse, emphasizing situational awareness—such as knowing when to flag suspicious data—and encouraging staff to report any concerns immediately.
Action Steps:- Ongoing Training: Conduct mandatory annual training for all investment professionals and compliance officers on the latest SEC regulations, case studies, and internal policies related to MNPI.
- Role-Based Learning: Tailor training sessions for different roles within the organization, such as portfolio managers, data analysts, and compliance staff, to ensure they understand how MNPI could manifest in their specific tasks.
- Hotlines for Reporting: Establish confidential reporting mechanisms (e.g., compliance hotlines) that allow employees to flag potential MNPI concerns without fear of retaliation.
- Documentation and Record-Keeping Maintaining detailed records of data acquisition and decision-making processes is crucial for proving regulatory compliance. In the event of an investigation, hedge funds must demonstrate that they have implemented sufficient safeguards to avoid MNPI. Clear documentation also allows firms to track the efficacy of their policies and refine them over time.
Action Steps:- Data Lineage Documentation: Keep detailed records showing the origin of all datasets, the review process they underwent, and the decisions made about their usage.
- Decision Logs: Maintain logs of all investment decisions made based on alternative data, including which datasets were used and why they were deemed free of MNPI.
- Regulatory Filings: Proactively file relevant disclosures with the SEC to demonstrate transparency in data usage.
Case Studies of MNPI Violations
- Nejat Seyhun’s Case: A hedge fund manager was penalized for using MNPI obtained from a research firm, leading to an insider trading scandal. The case underscores the importance of due diligence in vetting research and data providers. Compliance teams need to ensure that alternative data sources have not inadvertently gathered MNPI, especially from companies with restricted access to corporate insiders.
- Marwood Group Incident: In another enforcement action, the SEC fined Marwood Group for trading based on non-public information received from government employees, demonstrating how sensitive information can inadvertently flow into investment decisions, making it critical for compliance teams to monitor data flows and establish clear policies prohibiting the use of MNPI from any governmental or corporate insiders.
Expert Opinions on Mitigating MNPI Risks
Legal and compliance experts emphasize the need for hedge funds to invest in advanced compliance infrastructure and build cross-functional teams combining compliance officers, legal advisors, and data scientists. Jane Norberg, former Chief of the SEC’s Office of the Whistleblower, notes that firms should be more proactive in self-reporting compliance breaches. Self-reporting can lead to reduced penalties under the SEC’s enforcement guidelines.
David Blass, former Chief Counsel of the Investment Company Institute, highlights the importance of setting a “compliance-first” culture within firms. He suggests that hedge funds develop customized, industry-specific MNPI detection protocols that adapt to evolving data sources and regulatory frameworks.
Enhancing Alpha Generation with Alternative Data
Hedge funds have increasingly turned to alternative data as a critical tool for enhancing alpha generation, providing them with insights that go beyond traditional financial metrics. By leveraging non-traditional datasets such as satellite imagery, social media sentiment, and consumer transaction data, hedge funds can develop unique strategies that deliver a measurable competitive advantage. Below, we explore how hedge funds can effectively use alternative data to drive returns and enhance alpha, supported by research and specific analytical techniques.
Quantifying the Impact of Alternative Data on Returns
A 2024 study by J.P. Morgan found that hedge funds using alternative data experienced annual returns 3% higher than those relying solely on traditional data sources. Another report by Deloitte revealed that firms using alternative datasets saw a 10% increase in alpha generation over a five-year period, primarily due to the early identification of market trends and opportunities missed by conventional data.
Incorporating alternative data allows hedge funds to exploit new signals and react faster to changes in market dynamics. Eagle Alpha, an alternative data provider, reported that funds using their consumer transaction data were able to predict earnings surprises 2-3 weeks earlier than those using traditional earnings forecasts alone, allowing for more timely and informed trading decisions.
Methodologies for Leveraging Alternative Data
To fully unlock the potential of alternative data, hedge funds employ advanced analytical techniques that transform raw datasets into actionable investment signals. Here are a few key methodologies:
- Natural Language Processing (NLP) for Sentiment Analysis: Social media sentiment analysis, powered by NLP, allows hedge funds to gauge market sentiment on a real-time basis. Platforms like Twitter and Reddit offer a wealth of user-generated content that can signal shifts in public perception, which can be predictive of stock price movements. Research by RavenPack shows that stocks receiving a sudden increase in negative sentiment on social media underperform the broader market by 2.5% over the next month.
Example: Hedge funds can scan Twitter for negative sentiment spikes related to specific companies. By integrating this with traditional metrics, they can hedge their positions or sell off holdings before major declines. - Geospatial Analytics: Satellite imagery can provide real-time tracking of retail foot traffic, oil storage levels, or even the construction progress of new infrastructure. Hedge funds use machine learning models to analyze these images and predict sector-specific performance. An MIT Sloan study demonstrated that funds using satellite data to monitor retail parking lots were able to predict earnings beats or misses with 85% accuracy.
Example: By tracking the number of cars in parking lots at major retail chains, hedge funds can gauge whether a company’s quarterly sales are likely to beat or miss analyst estimates, enabling them to adjust their positions ahead of earnings announcements. - Transaction Data for Revenue Forecasting: Consumer transaction data offers insights into real-time spending patterns, allowing hedge funds to estimate company performance before official reports are released.
Example: By analyzing transaction data from major retailers, a hedge fund could predict a sales surge in the e-commerce sector during the COVID-19 pandemic, capitalizing on stock price increases before broader market analysts identified the trend.
Addressing Challenges and Data Quality Concerns
While alternative data offers immense potential for alpha generation, challenges around data quality, integration, and regulatory compliance must be addressed. In particular, hedge funds must ensure that data is both reliable and legally obtained.
To mitigate these challenges, hedge funds must adopt the following practices:
- Data Cleaning and Validation: Automated tools can help ensure that datasets are free of errors and biases before analysis. Firms like Quandl and YipitData offer data cleaning services that standardize and validate data to improve accuracy.
- Legal Compliance: With regulations like GDPR and CCPA governing data privacy, funds must verify that all alternative data used comply with these standards. Hedge funds often work closely with legal teams to audit data vendors and ensure consent and compliance are maintained.
Predictive Insights from Alternative Data
Including alternative data in the analysis allows hedge funds to generate predictive insights. By analyzing large datasets, patterns, and trends that might predict market shifts are revealed.
For instance, consumer purchasing data can hint at broader economic trends, while transaction data unveils potential inflection points in company performance. Hedge funds leverage these predictive capabilities to anticipate market changes and adjust strategies accordingly.
Data-driven strategies result in improved alpha generation by allowing hedge funds to act proactively rather than reactively. Alternative data’s predictive power aligns with the aim of consistently achieving superior returns, underscoring its importance in modern investment decision-making. Find more details in rethinking alternative data in institutional investment.
Explore the Future of Alternative Data with Daloopa
At Daloopa, we offer insights into the evolving role of data in fund management, provide a detailed analysis of leading data providers, and help you navigate ethical considerations, all while enhancing alpha generation.Create a free Daloopa account or click here to learn more about how alternative data can support your strategies and explore the tools we offer to help you make informed decisions.