Artificial intelligence (AI) is revolutionizing the financial industry by enabling companies to analyze vast amounts of data faster and more accurately. As a result, many leading financial data companies are harnessing the power of AI to enhance their offerings and stay competitive. From AI-driven financial data analysis to advanced risk management solutions, these innovations are changing the landscape of financial services.
Multiple studies and reviews have analyzed the applications of AI in finance, ranging from automating routine tasks and providing deeper insights into market data to improving accuracy and speed. These studies identify that advancements are crucial for staying ahead in the fast-paced investment banking world and ensuring compliance and accuracy.
Drawing from the commonly reported shortcomings and constraints of data companies and their AI models, innovative solutions like Daloopa AI are being developed to better meet standards in investment banking analysis. The ability to adapt and respond to changes quickly can make a significant difference in today’s equity management landscape. This article examines offerings from leading data companies, common applications of AI in financial products, and their pitfalls.
Jump to:
- Leading Financial Data Companies and Their AI Innovations
- 7 Common AI Applications in Finance
- 5 Common Challenges of Using AI in Finance
- Addressing Ethical Considerations in Finance AI
- Unlock the Future of Financial Analysis with AI
Leading Financial Data Companies and Their AI Innovations
In this section, we explore how some leading financial data companies leverage advanced AI technologies to elevate their services and offerings. This article examines AI offerings from leading data companies, common applications of AI in financial products, and their pitfalls.
S&P Global and Advanced Analytics
S&P Global has integrated advanced analytics into its suite of services to improve financial forecasting and risk assessment among other functionalities. By utilizing AI-driven data analysis, S&P Global helps clients gain deeper insights into market trends and economic indicators. Their advanced capabilities enable more accurate financial modeling, aiding investors and businesses in making informed decisions.
Functionality Overview:
S&P’s AI-driven tools provide advanced analytics using machine learning algorithms, sophisticated data science operations, generative AI, and Natural Language Processing. Products offered by the company feature AI-powered functionalities such as information retrieval, personalization, search tools, platform creation, and other enterprise solutions.
S&P Global leverages cloud computing capabilities via Snowflake’s Cloud Data platform, allowing the creation of databases that are conveniently accessible. These databases can be integrated into systems, portals, and other financial applications through API integration facilitated by the tool. S&P’s AI models are trained on extensive historical data, ensuring that the predictions are grounded in real-world financial conditions.
Tests and Validation:
S&P Global’s advanced features have undergone rigorous testing to ensure their reliability and accuracy. Studies have confirmed the effectiveness and revealed the limitations of financial models built by S&P’s AI technologies. S&P Global’s analytics tools are also assessed using finance-specific benchmarks, including those that are developed by Kensho.
Use Cases and Applications:
- Gather data from structured and unstructured datasets to compile pitch books and financial and analytical reports
- Compute credit ratings using credit risk assessment features
- Obtain analytics-driven insights to optimize investment portfolios
- AI-driven analysis of news, social media, and other sources to gauge market sentiment
- Automate routine analytical tasks to improve efficiency and reduce human error
- Extract information from texts and tables and transform them into machine-readable formats
Identified Challenges:
- Requires stringent privacy controls to handle sensitive financial data
- Requires expertise in AI technologies to comprehend and analyze outputs
- The accuracy of analytics depends heavily on the quality of the underlying data
Bloomberg and Machine Learning
Bloomberg leverages machine learning to enhance the quality and speed of financial data delivery. By employing algorithms akin to ChatGPT’s models, Bloomberg can process vast amounts of financial information, delivering actionable insights faster than traditional methods.
Functionality Overview:
Machine learning algorithms help AI tools learn from an exhaustive supply of source data and produce effective models. BloombergGPT’s machine learning functionalities have been developed with advanced computational capabilities and are specifically designed for finance. It is powered by NVIDIA’s computational tools, Amazon’s AWS cloud computing capabilities, and adopts a Unigram model powered by PyTorch, Python’s open-source deep learning package,
Bloomberg’s white paper details its capabilities extensively. The tool has been trained over a large domain-specific data input from financial websites, company filings, news, and press releases spanning over forty years. The model is trained with fragmented or tokenized data. This accelerates the tool’s precision and computational power.
Tests and Validation:
Bloomberg’s AI capabilities have been comprehensively tested industry-wide. This research paper discusses Bloomberg Professional’s adeptness in the academic front- as a tool for teaching finance and economics.
The product has also been validated on existing finance-specific NLP benchmarks by Bloomberg, and other popular general-purpose benchmarks like BIG-bench Hard, knowledge assessments, reading comprehension, and linguistic tasks. In a general sense, the model performs well in financial tasks while meeting general NLP benchmarks.
Use Cases and Applications:
- Read earning reports and other documents and summarize them
- Generate drafts of routine market reports and summaries for clients including documents for securities and exchange commissions
- Charting Applications help visualize and combine data across different asset classes
- Worksheets, Bloomberg’s version of Excel geared at finance, can be used to work with indices, securities, multiple portfolios, and apply customized portfolios
- Extract crucial information from isolated periods or elements
- Document Search automates the searching of applications
- Earnings Transcript Summary helps extract necessary information for summarizing information on earnings
Identified Challenges:
- Data privacy concerns
- Reliability and accuracy concerns
- Tokens can be costly in real-world applications
- Lack of access to source data
Thomson Reuters and AI Integration
Bloomberg’s rival Thomson Reuters integrates artificial intelligence across its diverse product offerings to better serve legal, financial, and media sectors. AI enhances their data processing capabilities, ensuring more precise and timely information dissemination. This integration allows clients to leverage comprehensive datasets for regulatory compliance and investment decisions.
Functionality Overview:
Given the data-driven nature of fields like law and finance, Thomson Reuters’ AI features incorporate machine-learning algorithms, risk modeling capabilities, and surveillance programs. Their tools are trained on large datasets and are programmed with natural language processing for better integration. They are also focused on being human-centric by employing a “human in the loop” approach to interacting with the user.
Thomson Reuters’ generative AI technology, CoCounsel is geared for tax and accounting industries and provides a single, secure, user-friendly chat interface that could help financial analysts perform in-depth research.
Tests and Validation:
Resources like Thomson Reuters’ white paper study the performance of AI tools in the financial industry. The organization’s AI products are tested against multiple criteria including publicly available benchmarks and Thomson Reuters’ standards for financial operations.
Use Cases and Applications:
- Reuters Financial News provides access to financial data, news, and content that can easily be understood and integrated into financial operations
- The Checkpoint solutions offered by Thomson Reuters offer intuitive search capabilities and insights for professionals in finance
- Thomson Reuters’ ONESOURCE software automates tax and compliance processes
- The CS Professional Suite allows users to seamlessly integrate systems, share data, and process workflows in real time for accounting and financial needs
- Their other AI-driven tools assist in automating routine tasks, freeing up time for higher-level analysis
Identified Challenges:
- Inherited biases from trained datasets
- Risk of fraud, swindling, and other security concerns with generative AI
- Lack of transparency in their decision-making processes
- The requirement of clean and robust data for smoother integration
Equifax and Predictive Analytics
Equifax utilizes predictive analytics to improve credit scoring and fraud detection. According to the company, nearly 80% of its operations are now handled by artificial intelligence. By analyzing vast datasets, Equifax.ai predicts consumer behavior and credit risks more accurately. This allows lenders to make informed decisions, reducing default rates and improving overall financial stability.
Functionality Overview:
Using AI techniques like deep analytics, neural networks, gradient-boosted machines, random forests, and machine learning, Equifax’s AI models can identify unusual patterns that may indicate fraudulent activity, help compute credit risks, and perform other operations. Equifax’s AI capabilities utilize the Equifax Cloud™ functionalities that provide in-built access to multi-data assets, real-time insights, and other embedded AI capabilities.
Equifax’s OneScore model provides a comprehensive overview of credit insights and credit reports to capture a company’s history beyond the information found in traditional credit reports. With more than 90 approved AI patents to its name, the agency is integrating more and more artificial intelligence functionalities into its products.
Tests and Validation:
Following the cybersecurity incident in 2017, Equifax and other top financial data companies have been vehemently scrutinized for their big data management practices. Many academic studies attempt to break down the causes and provide safety precautions to mitigate such security incidents. With the evolution of AI, and its extensive use in the financial world, such studies and tests are crucial for investment banking analysts and investors.
Use Cases and Applications:
- Generating credit reports and analyzing a company’s financial standing
- Real-time market insights
- Fraud prevention measures to minimize data breaches and identify potential fraudulent activities
- Access to a large collection of data from over 800 million consumers and businesses
- Credit and demographic data and services
Identified Challenges:
- Increased security concerns after the 2017 data breach
- Excessive dependence on AI functionalities
- Possible biases
7 Common AI Applications in Finance
In this brief overview, we discuss some common applications of artificial intelligence in financial services and analyses.
1. Real-Time Analytics and Decision Support
Financial markets demand up-to-the-minute data to make informed decisions. AI-driven real-time analytics process vast amounts of financial data, providing actionable insights instantly. Tools that leverage machine learning can be used to analyze market trends, predict stock prices, and detect fraudulent activities. This agility ensures better risk management and helps capitalize on emerging opportunities.
2. Automated Financial Reporting
AI-powered tools automate financial reporting processes, ensuring compliance and reducing manual errors. Technologies like natural language processing (NLP) can convert raw data into structured reports, summarizing performance metrics and financial statements. This article from ScienceDirect explores the use of AI in streamlining financial operations, particularly in data reporting.
3. Risk Management and Compliance
Predictive models are used to forecast market trends and manage portfolio risks effectively in equity management. These AI-driven tools provide real-time monitoring, flagging any deviations from compliance norms. Some fintech companies have also implemented AI to manage credit risks better. By evaluating creditworthiness through AI, financial institutions can make more informed lending decisions, reducing the probability of defaults.
4. Fraud Detection and Cybersecurity
AI-based systems analyze transaction patterns to detect anomalies that may indicate fraudulent activities. Machine learning models build profiles of typical behavior and spot deviations instantly, flagging suspicious behavior in real time. This lets users take prompt corrective actions, helping build confidence among customers and stakeholders.
5. Human-centric Chatbots
AI-powered chatbots can provide instant assistance, handling a variety of queries from account information to transaction details. They use natural language processing to understand queries and provide appropriate responses. These chatbots are capable of offering personalized recommendations based on customer interactions and previous data.
6. Investment Strategies and Robo-Advisors
Robo-advisors utilize algorithms to provide financial planning services with minimal human intervention. This technology offers personalized investment strategies based on individual risk tolerance and financial goals and offers automated portfolio management capabilities.
7. AI-powered Cloud Computing
Data is an important aspect of AI-driven models and requires proper management and storage. AI features now come embedded into these cloud computing platforms allowing access to tools essential for financial data management and handling large volumes of data. Financial analysts can derive actionable insights from complex datasets quickly.
5 Common Challenges of Using AI in Finance
In the following section, we briefly discuss some of the identified pitfalls of adopting AI in the financial and investment banking realm.
1. Integration with Existing Systems
Challenge:
Integrating AI solutions with existing financial systems can be costly, time-consuming, and complex. Legacy systems may need to be updated or designed to handle advanced AI algorithms, leading to compatibility issues.
Moreover, integrating new AI tools involves a “deployment lag time” and can significantly impact existing workflows and processes. This leads to data silos, where data is isolated within different departments or systems of the same organization.
Possible Solutions:
Integrating AI requires better training processes, a unified view of data, and integration of intelligent systems. Daloopa AI addresses this by providing a comprehensive migration guide document that walks users through the process of integrating its AI tools into existing financial models.
This challenge can be further addressed by building intelligent systems tested against industry benchmarks after being trained using complex algorithms and labeled data. Companies are also adopting modular approaches to integration, allowing AI tools to interact with legacy systems through intermediary layers or APIs.
2. Inherent Biases
Challenge:
Algorithmic bias is a prominent challenge faced within AI systems. In financial services, it can have negative implications on fairness and outcomes, proliferating into crucial management decisions.
Possible Solutions:
Data companies have attempted to address this by training models in a wide range of inclusive data representations reflective of diverse demographics and scenarios in finance. Sophisticated ensemble models may also be used to minimize such biases and promote equitable decision-making processes prioritizing diversity, inclusiveness, and fairness.
3. Data Quality and Security Issues
Challenge:
A recent study by Deloitte confirmed that data quality issues are among the top three reasons AI falls short of meeting financial objectives. AI systems rely on high-quality data to function effectively. In finance, data quality issues can arise from inaccuracies, inconsistencies, or incomplete data. Poor data quality can lead to erroneous predictions and analyses.
Financial data from different sources may be inconsistent, affecting the accuracy of AI models. Historical data may contain biases that can lead to biased AI outcomes, impacting decision-making processes.
Possible Solutions:
Companies are investing in data cleansing and preprocessing tools to ensure data accuracy and consistency. For instance, Goldman Sachs has implemented advanced data quality frameworks to ensure the reliability of their AI models.
Techniques such as adversarial debiasing and fairness-aware modeling are also being employed to address biases in AI systems. Daloopa AI allows maximum transparency for users to identify source data and execute operations accordingly.
4. Regulatory Compliance
Challenge:
The financial industry is highly regulated, and AI implementations must comply with various regulatory requirements. Ensuring that AI systems adhere to these regulations while maintaining their effectiveness can be challenging.
Financial regulations are continuously evolving, and keeping AI systems compliant with the latest regulations like the Fair Credit Reporting Act (FCRA) requires ongoing updates. Most regulations often require transparency in decision-making processes, which can be difficult to achieve with complex AI models.
Possible Solutions:
Companies are leveraging Regulatory Technology (RegTech) to manage compliance more effectively. RegTech solutions help automate compliance processes and ensure adherence to regulations. For example, HSBC has adopted RegTech tools to streamline compliance monitoring and reporting.
5. Technical Challenges
Challenge:
The “black box” issue is an inherent concern in AI models, particularly in finance where details of intrinsic operations are crucial. This lack of transparency undermines the accountability and trust within the industry. Other technical issues include slow response times and high development requirements.
Possible Solutions:
The adoption of explainable AI and improvements in model interpretability can enhance reliance on complex algorithms. Techniques like distributed real-time computing and cloud-based AI services are being employed to scale AI models effectively. Implementing high-performance computing frameworks and optimizing algorithms for speed can address real-time processing requirements.
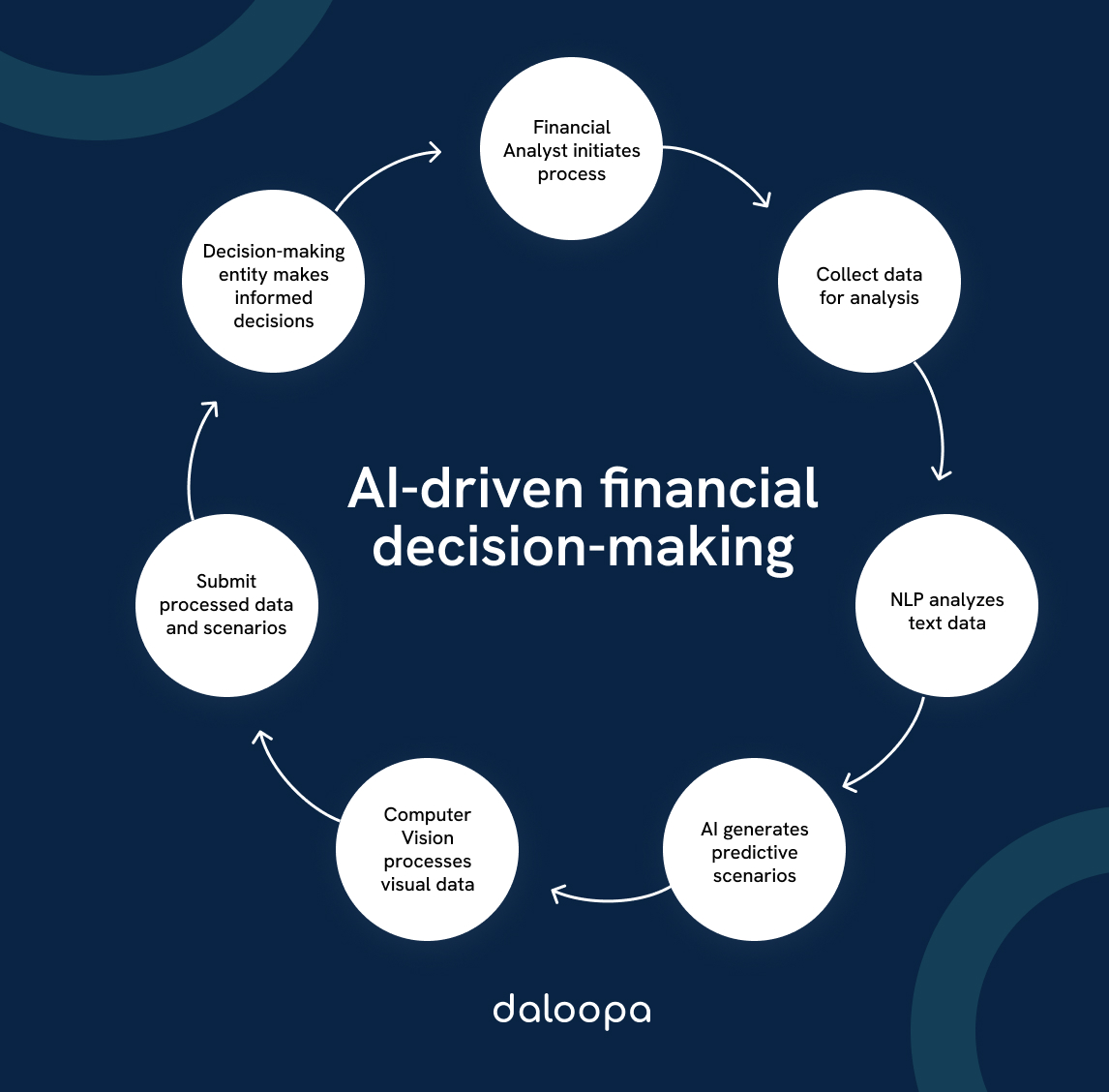
Addressing Ethical Considerations in Finance AI
An EY survey revealed that nearly two-thirds of CEOs understand the importance of addressing ethical considerations of using AI in finance. Critical challenges and ethical considerations when integrating AI into financial services include managing biases and building trust, ensuring data privacy while adhering to regulatory requirements, and employing responsible AI for financial decision-making.
Addressing Bias and Trust Issues
Bias in AI systems can lead to unfair and discriminatory outcomes in financial services. Algorithms may inadvertently favor certain demographics over others due to biased training data. This lack of fairness erodes trust in these systems.
To address this bias, we must implement rigorous data auditing practices and transparency measures. By ensuring diverse training data sets, we can limit the chances of biased outcomes. Furthermore, the use of ensemble models and regular evaluation of AI models for performance and fairness can help sustain public trust and integrity.
A study submitted to ResearchGate explores how AI technologies can be integrated into current and evolving regulations in the finance management realm. It broadly analyzes the use of machine learning algorithms to enhance regulatory compliance in AI-driven financial tools.
Data Privacy and Regulatory Compliance
Financial institutions handle sensitive information, making data privacy crucial. AI systems must comply with stringent regulations such as GDPR in Europe and CCPA in California. Non-compliance can result in significant fines and reputational harm.
Effective data anonymization and encryption methods are essential practices for protecting customer data. Additionally, establishing robust data governance frameworks and differential privacy techniques ensures that we handle data in line with privacy laws and standards. Regular audits and updates to these frameworks help us stay compliant with evolving regulations.
Responsible AI for Financial Decision-Making
Applying AI responsibly involves understanding its impact on financial decision-making processes. AI should enhance, not replace, human judgment in critical financial decisions. This involves setting predefined ethical guidelines and practices.
Employing explainable AI techniques to make AI decisions transparent, establishing modeling guidelines and standards, and human oversight can enhance the accountability factor of these tools. By doing so, we provide stakeholders with clarity on how decisions are made. Combining human oversight with AI capabilities ensures a balanced, ethical approach to financial decision-making, ultimately benefiting all parties involved.
Unlock the Future of Financial Analysis with AI
Are you struggling with data overload or finding it challenging to make quick, accurate financial decisions? AI is the new norm, and financial data companies are contending to revolutionize financial analysis and decision-making with AI-powered tools. Industry leaders like Bloomberg and S&P Global are transforming financial analysis with AI-powered tools, and you can too. Daloopa’s advanced AI capabilities are designed to address common challenges faced by analysts—whether it’s managing vast amounts of data, accelerated decision-making, or seamlessly integrating AI into your existing workflows. Don’t fall behind—join the ranks of top analysts who are already using AI in their strategies. Create a free Daloopa account today and explore how our advanced AI capabilities can transform your financial analysis.